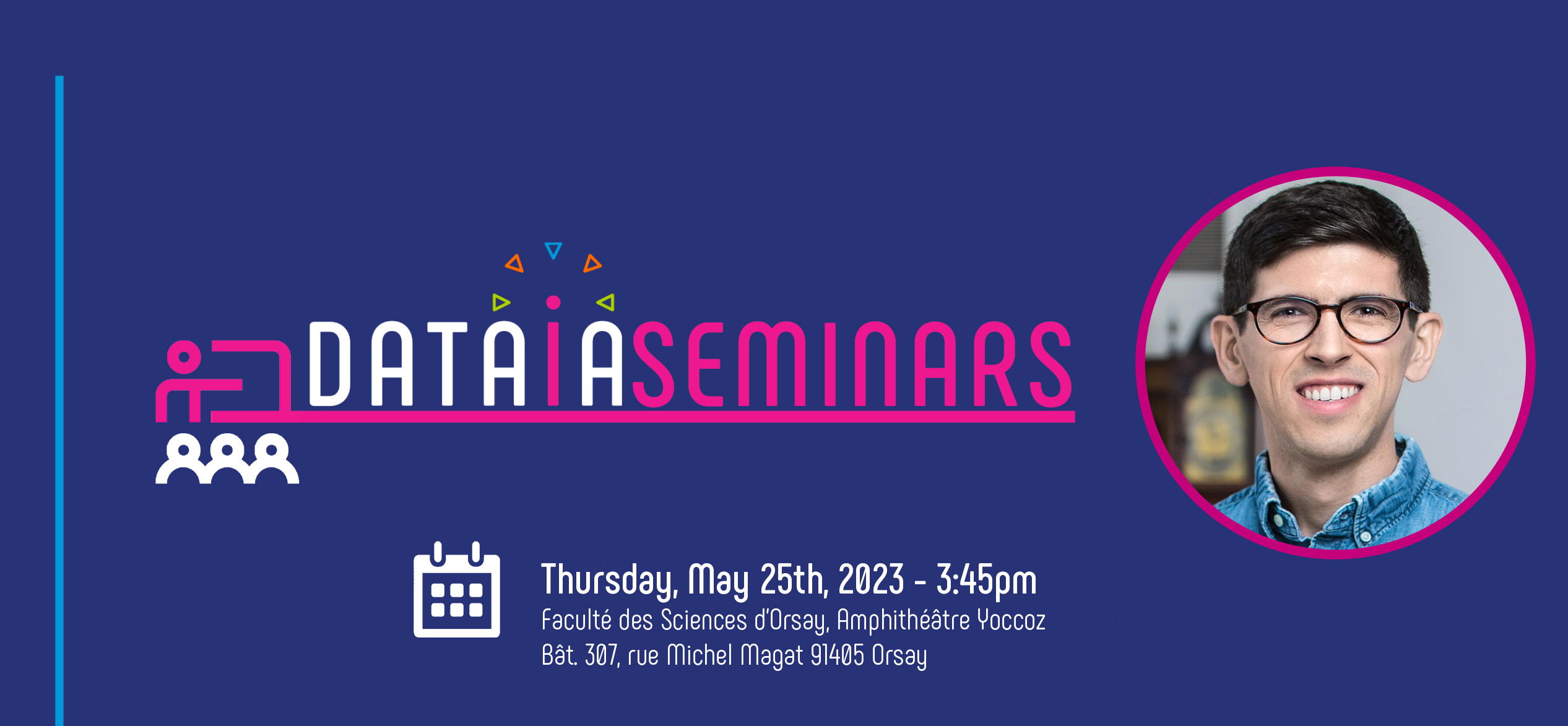
We are pleased to welcome Jonathan Niles-Weed, Assistant Professor of Mathematics and Data Science at the Courant Institute of Mathematical Sciences and Center for Data Science (New York University), who will present his work on the topic "Optimal Transport Map Estimation in General Function Spaces".
Abstract: We consider the problem of estimating the optimal transport map between a (fixed) source distribution P and an unknown target distribution Q, based on samples of Q. The estimation of such optimal transport maps has become increasingly relevant in modern statistical applications, such as generative modeling. Currently, estimation rates are known only in a few cases (e.g., when P and Q have bounded densities above and below and when the transport map is in a Hölder class), which are often not considered in practice. We present a unified methodology for obtaining optimal transport map estimation rates in general functional spaces. Our assumptions are much weaker than those in the literature: we only require that the source measure P satisfies a Poincaré inequality and that the optimal map is the gradient of a smooth convex function that lies in a space whose metric entropy can be controlled. In one particular case, we find known estimation rates for bounded densities and Hölder transport maps, but we also obtain almost clear results in many settings not covered by previous work. For example, we provide the first statistical estimation rates when P is the normal distribution and the transport map is given by a shallow neural network of infinite width.
- The seminar will be held on Thursday, May 25th, 2023 at the Faculty of Sciences of Orsay (Amphi Yoccoz, build. 307) at 3:45pm. It will be followed by a break.
- Registration is mandatory subject to availability!
Don't miss the announcement of a new DATAIA seminar!
Sign up for our seminar mailing list by clicking here.