June 27 to July 8, 2022 - Thematic Quarter - Artificial Intelligence (AI) for Signal and Image Processing Program
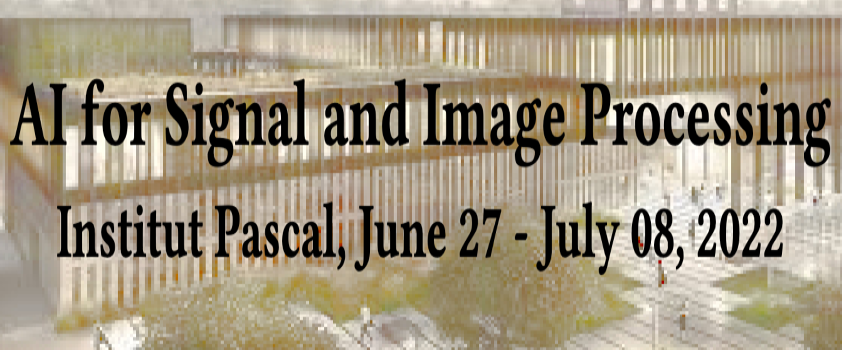
The 2-week program aims to provide an opportunity for researchers and especially young researchers (e.g., PhD students, postdoctoral fellows) to learn the underlying theory and concepts discussed in the program, to share their knowledge, and to develop new collaborations.
The program will be dedicated to :
- 1. Signal processing theory and methods ;
- 2. The empirical evaluation of algorithms on open data sets, i.e., benchmarks ;
- 3. Data challenges and coding sprints for developing open-source software ;
- 4. Journal clubs aiming to survey the literature and make recommendations on specific problems.
A non-exhaustive list of scientific research topics was previously sketched out during the IPa program “teasing day”, held in September 2021.
Scientific and organizing committees
Scientific Committee : E. Chouzenoux (Inria Paris-Saclay), A. Desolneux (ENS Paris-Saclay), A. Gramfort (Inria Paris-Saclay), A. Kazeykina (Paris-Saclay University), M. Kowlaski (Paris-Saclay University).
Organizating Committee : P. Ciuciu (CEA), F. Pascal (CentraleSupélec), C. Soussen (CentraleSupélec), B. Thirion (Inria Paris-Saclay).
At the occasion of this thematic program, a conference on the theme "Rapture of the deep: highs and lows of sparsity in a world of depths" will be held at the Institut Pascal and will be open to the general public. This seminar will be animated by Rémi GRIBONVAL, INRIA Research Director.
Abstract
Attempting to promote sparsity in deep networks is natural to control their complexity, and can be expected to bring other benefits in terms of statistical significance or explainability. Yet, while sparsity-promoting regularizers are well under-stood in linear inverse problems, much less is known in deeper contexts, linear or not. We show that, in contrast to the linear case, even the simple bilinear setting leads to surprises: ℓ1 regularization does not always lead to sparsity [1], and optimization with fixed support can be NP-hard [2]. We nevertheless identify families of supports for which this optimization becomes easy [2] and well-posed [3], and exploit this to derive an algorithm able to recover multilayer sparse matrix factorizations with certain prescribed (butterfly) supports at a cost proportional to the size on the approximated matrix [4,5]. Behind much of the observed phenomena are intrinsic scaling ambiguities in the parameterization of deep linear networks, which are also present in ReLU networks. We conclude with a scaling invariant embedding of such networks [6], which can be used to analyze the identifiability of (the equivalence class of) parameters of ReLU networks from their realization.
[1] A. Benichoux, E. Vincent, R. Gribonval, A fundamental pitfall in blind deconvolution with sparse and shift-invariant priors, Proc. ICASSP 2013.
[2] Q.T. Le, E. Riccietti, R. Gribonval, Spurious Valleys, Spurious Minima and NP-hardness of Sparse Matrix Factorization With Fixed Support, 2021, arXiv:2112.00386.
[3] L. Zheng, E. Riccietti, R. Gribonval, Identifiability in Two-Layer Sparse Matrix Factorization, 2021, arXiv:2110.01235.
[4] Q.T. Le, L. Zheng, E. Riccietti, R. Gribonval, Fast learning of fast transforms, with guarantees, Proc. ICASSP 2022
[5] L. Zheng, E. Riccietti, R. Gribonval, Efficient Identification of Butterfly Sparse Matrix Factorizations, 2022, arXiv:2110.01230
[6] P. Stock, R. Gribonval, An Embedding of ReLU Networks and an Analysis of their Identifiability, to appear in Constructive Approximation
See you on July 5th at 5:30pm, at the Institut Pascal !