Le Séminaire Palaisien | Machine Learning and Statistics
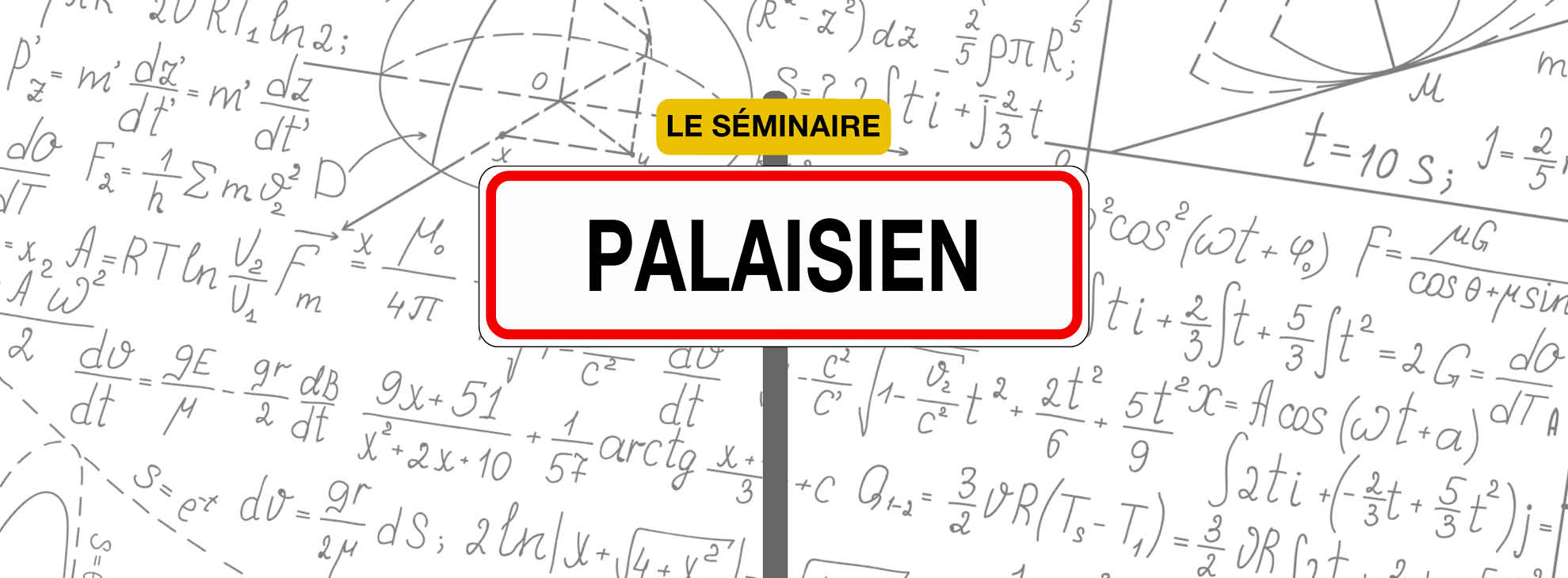
Each seminar session is divided into 2 scientific presentations of 40 minutes each: 30 minutes of presentation and 10 minutes of questions, followed by a coffee break.
Hicham Janati (PhD student in the Parietal team, Inria) and Rainer Dyckerhoff (professor at the University of Cologne), will lead the session of the 11th February.
Comparing data defined over space and time is notoriously hard, because it involves quantifying both spatial and temporal variability, while at the same time taking into account the chronological structure of data. For instance, MEG and EEG source estimates provide brain activity maps in a millimetre / millisecond resolution with significant spatio-temporal differences. Evaluating how two different MEG time series are close to each other is thus far from trivial. This work is a first step towards that goal. Dynamic Time Warping (DTW) computes an optimal alignment between time series in agreement with the chronological order, but is inherently blind to spatial shifts. In this paper, we propose Spatio-Temporal Alignments (STA), a new differentiable formulation of DTW, in which spatial differences between time samples are accounted for using regularized optimal transport (OT). Our temporal alignments are handled through a smooth variant of DTW called soft-DTW, for which we prove a new property: soft-DTW increases quadratically with time shifts. The cost matrix within soft-DTW that we use is computed using unbalanced Optimal transport. Experiments on brain imaging data confirm our theoretical findings and illustrate the effectiveness of STA as a dissimilarity for spatio-temporal data.
Depth is a concept that measures the centrality of a point in a given data cloud x_1, x_2,..., x_n in ℝ^d or in a given probability distribution on ℝ^d. For statistical applications it is desirable that with increasing sample size the empirical depth as well as the empirical central regions converge almost surely to their population counterparts.
After a short introduction in the general concept of depth we first discuss the continuity properties of depths and central regions. The main part of the talk is concerned with the connections between different types of convergence. We give conditions under which the pointwise (resp. uniform) convergence of the data depth implies the pointwise (resp. uniform) convergence of the central regions in the Hausdorff metric as well as conditions under which the reverse implications hold. Further, we demonstrate that under relative weak conditions the pointwise convergence of the data depth (resp. central regions) is equivalent to the uniform convergence of the data depth (resp. central regions). Finally, we illustrate these results by applying them to special notions of data depth that have been proposed in the literature.
The seminar will be followed by a coffee break.
Registration free but mandatory within the limit of available seats.
For security reasons, no access to the conference room for unregistered participants