« Le Séminaire Palaisien » | Vincent Divol and Alain Durmus on machine learning and statistics
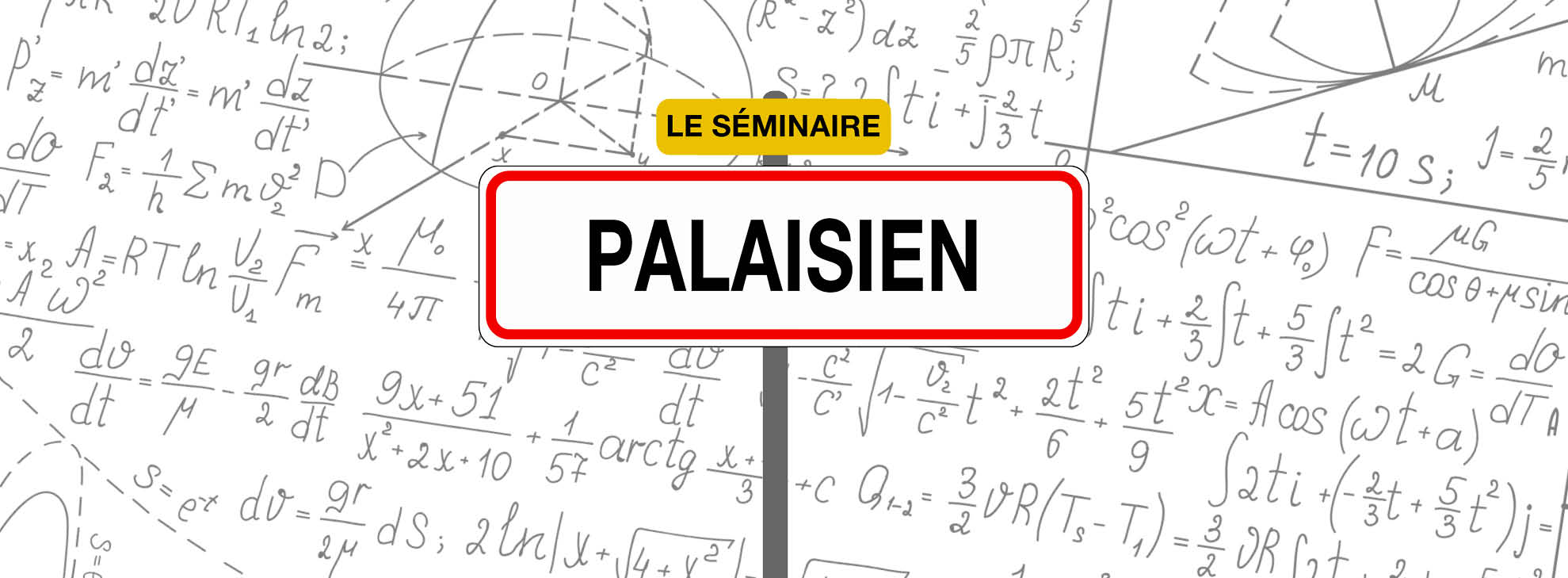
Each seminar session is divided into 2 scientific presentations of 40 minutes each: 30 minutes of presentation and 10 minutes of questions.
Vincent Divol (Inria) and Alain Durmus (ENS Paris-Saclay) will lead the session of January 2021.
Density estimation is one of the most classical problem in nonparametric statistics: given i.i.d. samples from a distribution mu on R^D, the goal is to reconstruct the underlying density (say for instance for the L_p norm). This problem is known to become untractable in high dimension D >> 1. We propose to overcome this issue by assuming that the distribution mu is actually supported around a low dimensional unknown shape M, of dimension d << D. After showing that this problem is degenerate for a large class of standard losses (L_p, total variation, etc.), we focus on the Wasserstein loss, for which we build a minimax estimator, based on kernel density estimation, whose rate of convergence depends on d, and on the regularity of the underlying density, but not on the ambient dimension D.
Markov Chain Monte Carlo (MCMC) is a class of algorithms to sample complex and high-dimensional probability distributions. The Metropolis-Hastings (MH) algorithm, the workhorse of MCMC, provides a simple recipe to construct reversible Markov kernels. Reversibility is a tractable property which implies a less tractable but essential property here, invariance. Reversibility is however not necessarily desirable when considering performance. This has prompted recent interest in designing kernels breaking this property. At the same time, an active stream of research has focused on the design of novel versions of the MH kernel, some nonreversible, relying on the use of complex invertible deterministic transforms. While standard implementations of the MH kernel are well understood, aforementioned developments have not received the same systematic treatment to ensure their validity. In this talk, we will introduce develop general tools to ensure that a class of nonreversible Markov kernels, possibly relying on complex transforms, has the desired invariance property and lead to convergent algorithms. This leads to a set of simple and practically verifiable conditions.