« Seminar Le Palaisien » | Marylou Gabrié & Gaël Varoquaux
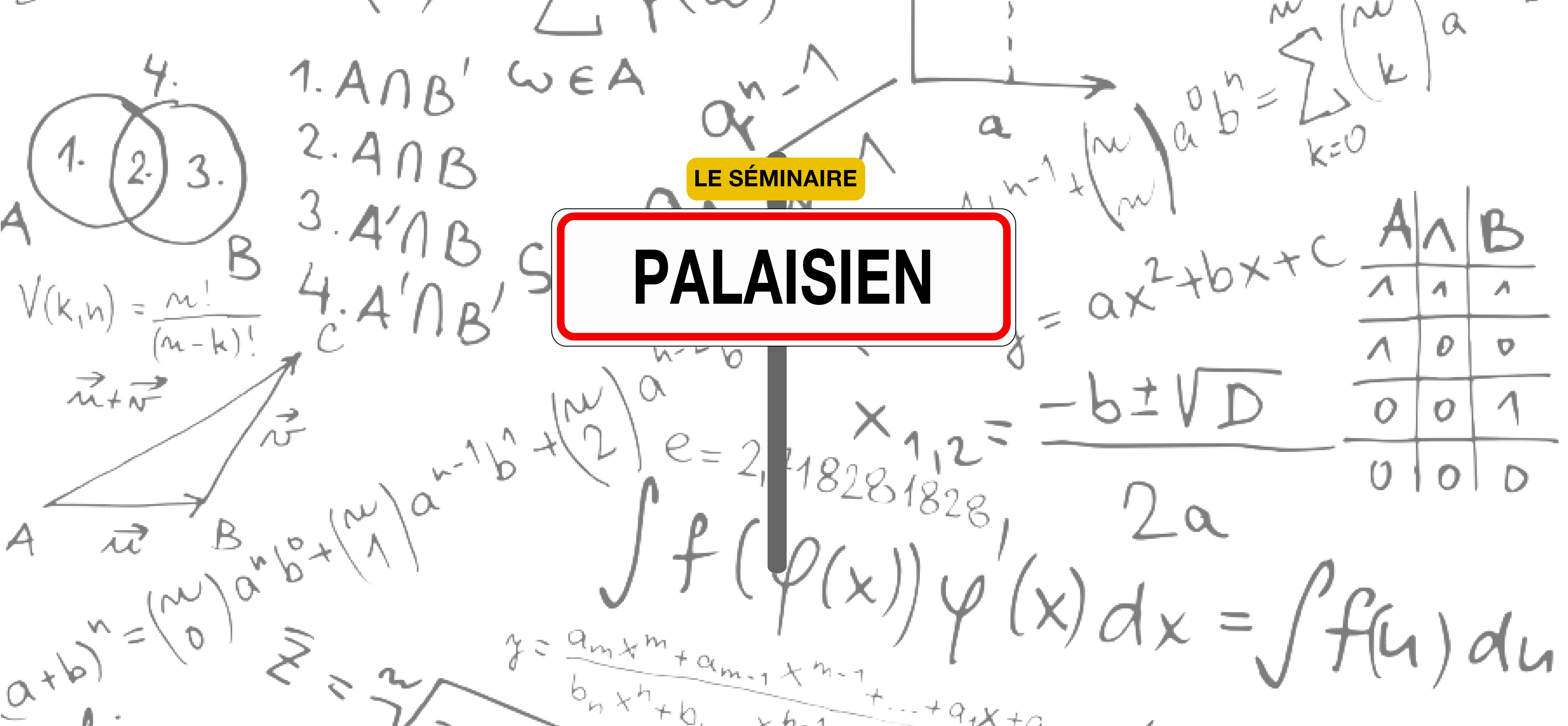
Each seminar session is divided into two scientific presentations of 40 minutes each: 30 minutes of presentation and 10 minutes of questions.
Marylou Gabrié et Gaël Varoquaux will moderate the March 2023 session.
Registration is free but mandatory, subject to availability. A sandwich basket is offered.
Abstract: deep generative models parameterize highly flexible families of distributions capable of fitting complex image or text data sets. These models provide independent samples of high complex distributions at negligible costs. On the other hand, accurate sampling of a target distribution, such as a Bayesian posterior, is generally difficult: either due to dimensionality, multi-modality, poor conditioning, or a combination of these factors. In this talk, I will review recent work that attempts to improve on traditional inference and learning-based sampling algorithms. In particular, I will present flowMC, an adaptive MCMC with normalization flows, as well as initial applications and remaining challenges.
Abstract: statistical learning relies on regularities in data, exploiting similarities between observations or the smoothness of the underlying process. But these regularities, similarities or regularity are difficult to capture in relational data. Observations are accompanied by attributes of different nature: age, height, address. The observations themselves can be of a different nature, reflecting a different granularity of information. For example, studying the housing market may require gathering information about sales, properties, buyers, and various administrative divisions of cities and states.
Faced with such complex relational data, the common practice is to manually transform it into a vector space, with a lot of manual work to make the data as regular as possible: SQL joins and aggregations between tables, entity normalization (correcting typos), imputing missing values. I will present progress in rethinking the data science process to avoid these manual operations. The use of flexible learners rather than parametric models removes the need for fancy imputation [1]. Character-level machine learning removes the need for entity normalization, although the analytical question must be reformulated with a nonparametric model [2,3]. Finally, the information of a complete database, with objects of different nature and varying attributes, can be expressed in a vector space that captures this information by expressing the relational model as a graph and adapting knowledge graph integration techniques [4]. As a result, we provide vectors summarizing all numerical and relational information of wikipedia for millions of entities: cities, people, companies, books: https://soda-inria.github.io/ken_embeddings/.
[1] Marine Le Morvan, Julie Josse, Erwan Scornet, & Gaël Varoquaux, (2021). What’s a good imputation to predict with missing values?. Advances in Neural Information Processing Systems, 34, 11530-11540.
[2] Patricio Cerda, and Gaël Varoquaux. Encoding high-cardinality string categorical variables. IEEE Transactions on Knowledge and Data Engineering (2020).
[3] Alexis Cvetkov-Iliev, Alexandre Allauzen, and Gaël Varoquaux. Analytics on Non-Normalized Data Sources: more Learning, rather than more Cleaning. IEEE Access 10 (2022): 42420-42431.
[4] Alexis Cvetkov-Iliev, Alexandre Allauzen, and Gaël Varoquaux. <a href="https://hal.science/hal-03848124">Relational data embeddings for feature enrichment with background information.</a> Machine Learning (2023): 1-34.