DATAIA Seminar | « Machine learning and causal inference: a two-way road » - Uri Shalit
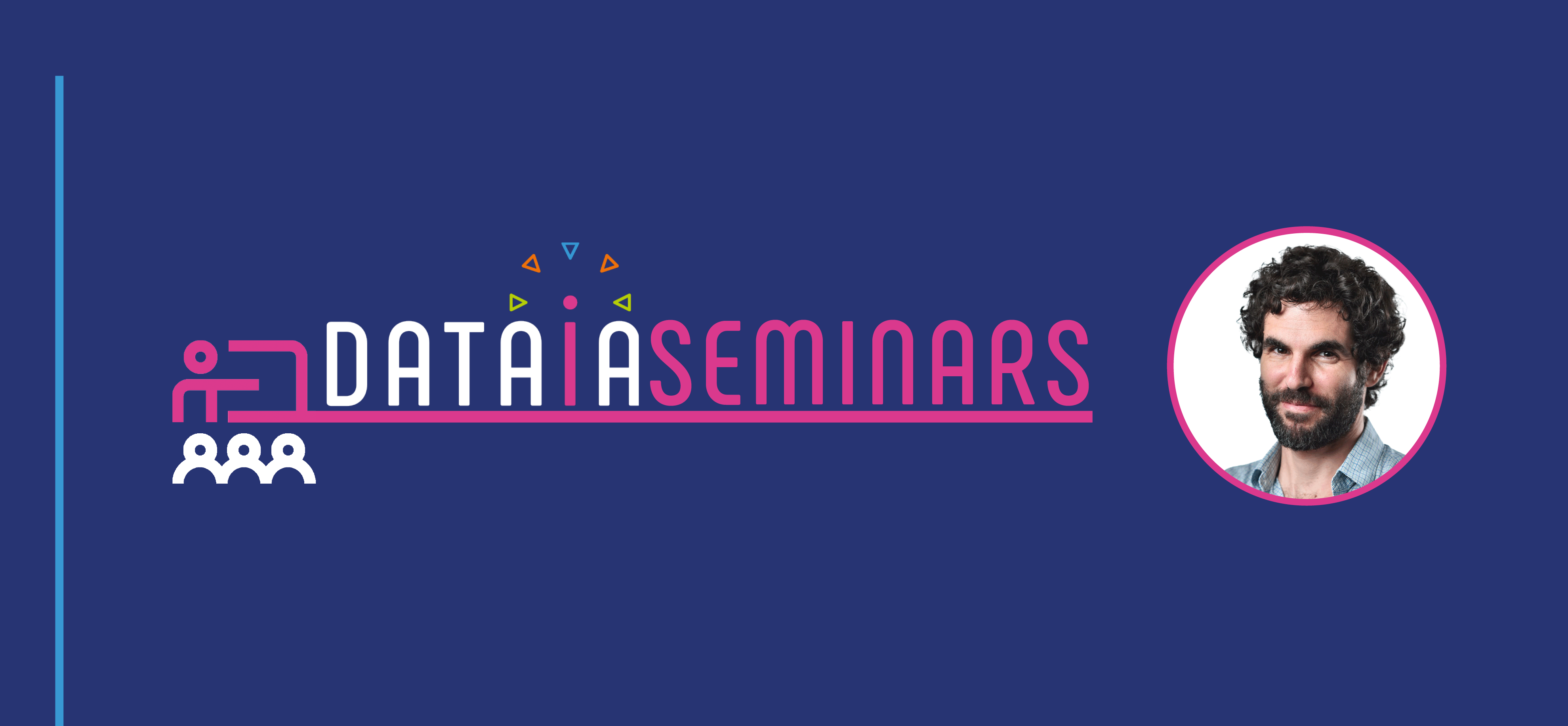
Uri Shalit (Israel Institute of Technology) is leading the DATAIA seminar of January 23, on « Machine learning and causal inference: a two-way road ».
This talk will have two parts. In the first we will discuss how and when can deep learning methods be applied to learning individual-level causal effects. We will then present a framework we developed for learning individualized treatment recommendations from observational health data, using data of tens of thousands of patients from a big health provider. In the second part we will show how we use ideas from the causal inference literature to address long standing problems in machine learning: off-policy evaluation in a partially observable Markov decision process (POMDP), and learning predictive models that are stable against distributional shifts.
Uri Shalit is a senior lecturer at the Technion - Israel Institute of Technology.
Previously, he was a postdoctoral researcher in Prof. David Sontag’s Clinical Machine Learning Lab in NYU and then MIT. He completed my PhD studies at the School of Computer Science & Engineering at The Hebrew University of Jerusalem, under the guidance of Prof. Gal Chechik and Prof. Daphna Weinshall. From 2011 to 2014 he was a recipient of Google's European Fellowship in Machine Learning.
His research focuses on two themes.
The first is applying machine learning to the field of healthcare, especially in terms of providing physicians with decision support tools based on big health data.
The second is the intersection of machine learning and causal inference, with a focus on using deep-learning methods for causal inference.
The seminar will take place on January 23 from 3pm to 4pm at the Inria-Saclay Center - Alan Turing building - Amphitheatre Sophie Germain.
It will be followed by a coffee break.
Registration free but mandatory within the limit of available seats.
For security reasons, no access to the conference room for unregistered participants.