WORKSHOP | "Mathematical foundations of AI" - 4th edition
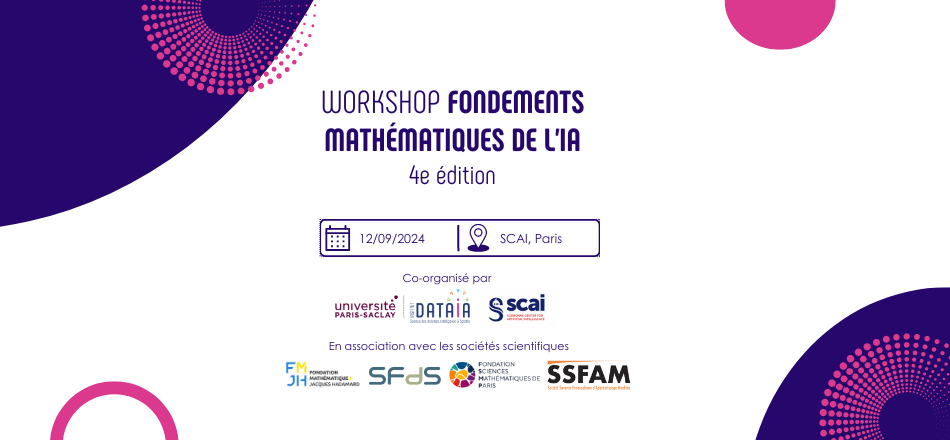
Registration closed!
The "Mathematical Foundations of AI" day, jointly organized by the DATAIA Institute and SCAI, in association with the scientific societies: the Fondation Mathématique Jacques Hadamard (FMJH), the Fondation Sciences Mathématiques de Paris-FSMP, the MALIA group of the Société Française de Statistique and the Société Savante Francophone d'Apprentissage Machine (SSFAM) aims to offer an overview of some promising research directions at the interface between statistical learning and AI.
This new edition will be devoted to the mathematics of risks. The day is devoted to presentations by renowned researchers and specialists in the field : A. Charpentier (UQAM, Montréal-Canada), S. Loisel (CNAM, Paris), O. Lopez (ENSAE, Paris), M. Thomas (ISFA, Lyon).
Organizing Committee
- Marianne Clausel (Univ. Lorraine)
- Emilie Chouzenoux (INRIA Saclay, DATAIA Institute)
Scientific Committee
- Ricardo Borsoi (CNRS, CRAN)
- Stéphane Chrétien (Univ. Lyon 2)
- Sylvain Le Corff (Sorbonne University)
- Myriam Tami (CentraleSupélec)
-
9am - 10am | Keynote 1 : A. Charpentier (UQAM, Montréal-Canada)
-
10am - 10:30am | Coffee break
-
10:30am - 11:30am | Keynote 2 : M. Thomas (ISFA, Lyon)
-
11:30am - 12:15pm | Short contributive talks (20')
Maxime Meloux (LIG, Grenoble Alpes University)
Clément Yvernes (UGA) -
12:15pm - 1:45pm | Lunch break
-
1:45pm - 2:45pm | Keynote 3 : S. Loisel (CNAM, Paris)
-
2:45pm - 3:30pm | Coffee break
-
3:30pm - 4:30pm | Keynote 4 : O. Lopez (ENSAE, Paris)
-
4:30pm - 5:30pm | Short contributive talks (20')
Mouad El-Bouchattaoui (MICS, CentraleSupélec & St Gobain)
Simon Weinberger (ERIC, Lyon 2 & ESSILOR)
Mouad El-Bouchattaoui (MICS, CentraleSupélec & St Gobain)
Random effects in longitudinal data through the lens of causal inference
Mixed effect modeling is a widely used technique incorporating random effects to capture individual-level variations and account for unobserved factors that may influence treatment response. By explicitly modeling these random effects, mixed effect models provide a framework for adjusting treatment effects based on latent variables. Interestingly, we establish a connection between mixed-effect modeling and causal inference. While mixed-effect models address unobservable heterogeneity by including random effects, one can focus on learning a representation of the unobserved variables and incorporating them into the estimation of Individual Treatment Effects (ITEs). This connection highlights the shared objective of both approaches: properly accounting for unobservable sources of heterogeneity to enhance the estimation of treatment effects. From a causal inference perspective, traditional methods often assume that all confounders are observed or attempt to infer the unobserved ones. Diverging from these approaches, we assume unobserved risk factors—adjustment variables that influence only the outcome sequence, not the treatment assignment, representing the sources of random effects. Building upon the foundation of the Dynamic Variational Autoencoder (DVAE) framework, we extend it to causal inference with the development and implementation of the Causal DVAE (CDVAE). Guided by theoretical results that validate the learned adjustment variables and establish generalization bounds for treatment effects, our model outperforms state-of-the-art methods.
We base our work on graphical statistics principles, using causal graphs to describe interactions between variables in a time-varying context. These graphs encode the data generation process and identify missing variables and their causal links to observed ones. We validated our methodology through extensive experiments, demonstrating the superiority of CDVAE in ITE estimation over existing baselines. This work emphasizes the importance of considering unobserved risk factors in causal inference and offers a powerful tool for researchers and practitioners dealing with complex, high-dimensional sequential data.
Maxime Meloux (LIG, Grenoble Alpes University)
Causal abstraction and interpretability in machine learning
Although the interpretability of machine learning systems is crucial to their transparency, reliability, legal compliance and user confidence, current interpretation methods face various problems of scope, globality and causality. A promising approach to addressing these issues comes from the fields of causal analysis and inference. In this talk, we propose a more precise definition of the notion of explicability, and argue that causal abstraction offers a general framework that meets this definition and is conducive to the explicability of neural networks. We then present the implementation of a testbed based on causal abstraction, enabling the evaluation of current and future explicability methods.
Simon WEINBERGER (ERIC, Lyon 2 & ESSILOR)
Reinforcement learning proposes a flexible framework that allows to tackle problems where data is gathered in a dynamic context: actions have an influence on future states. The classical reinforcement learning paradigm depends on a Markovian hypothesis, the observed states depend upon past states only through the last state and action. This condition may be too restrictive for real-world applications, as the dynamics may depend on past states. We get around this issue by augmenting the state space into a functional space, and we propose to use policies that depend on these states using functional regression models. We present an industrial application involving the automatic tint control of e-chromic frames, minimizing the number of user interactions. This particular policy takes action on an ordered set, using functional data estimated from successive Ambient Light Sensor (ALS) values, which are non-stationary. This is achieved using an extension of an existing ordinal model with functional covariates as a policy. The non-stationary ALS signal describing the state is handled by means of a wavelet functional basis. Finally, the policy is improved using policy gradient methods.
Clément Yvernes (UGA)
In causal analysis, knowledge of the causal graph underlying a system generally enables the desired effects to be identified. However, obtaining such graphs is often a complex challenge. In particularly difficult contexts, it is necessary to resort to abstractions that represent causality in a simplified way. While such abstractions are useful, they reduce the amount of information available, making causal reasoning more complex. This talk examines the different notions of identifiability that emerge when reasoning from causal abstractions, and the relationships that link them. Particular attention will be paid to SCGs, causal abstractions applied to time series, where specific conditions ensure the identification of effects.