« Le Séminaire Palaisien » | Florent Bouchard et Jaouad Mourtada sur l'apprentissage automatique et la statistique
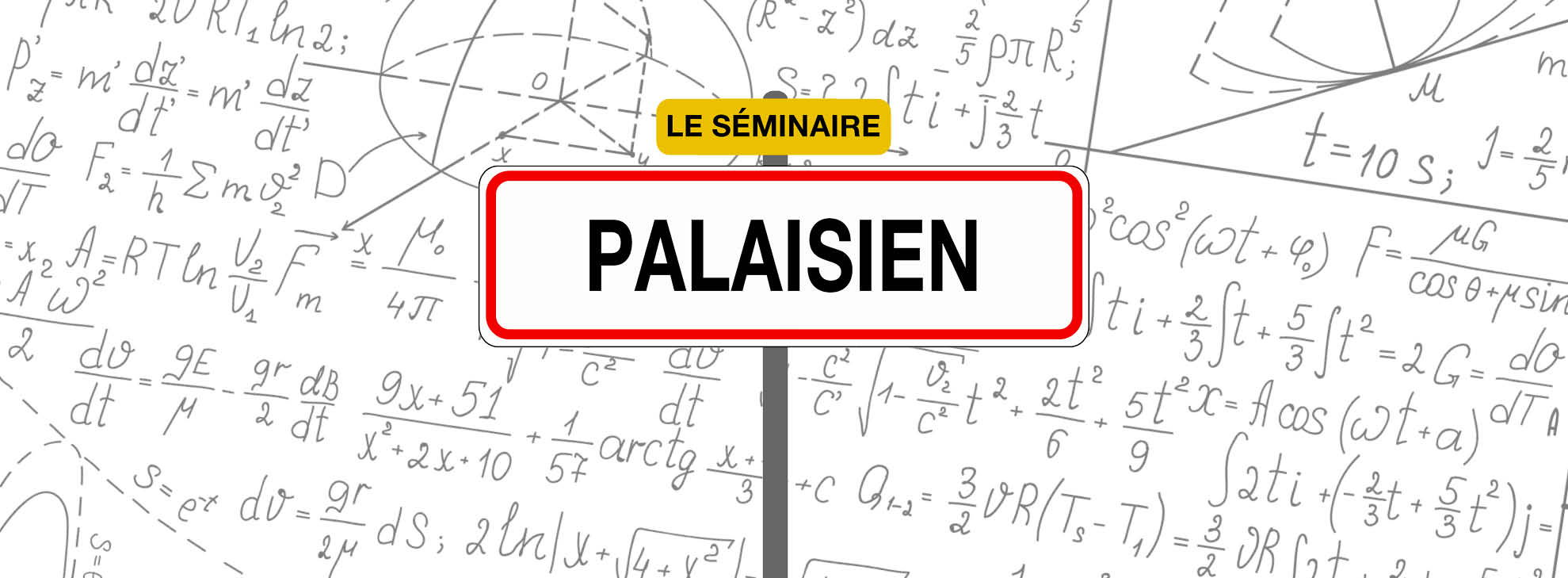
Chaque session du séminaire est divisée en deux présentations scientifiques de 40 minutes chacune : 30 minutes d’exposé et 10 minutes de questions.
Florent Bouchard (Université Savoie Mont Blanc) et Jaouad Mourtada (ENSAE) animeront la session de mars 2021.
In this presentation, Riemannian geometry for data analysis is introduced. In particular, it is applied on two specific statistical signal processing problems: blind source separation and low-rank structured covariance matrices. Blind source separation can be solved by jointly diagonalizing some covariance matrices. We show here how geometry can be exploited in order to provide original joint diagonalization criteria and ways to compare them theoretically. These results are illustrated with numerical experiments both on simulated and real data. Concerning low-rank structured covariance matrices, an intrinsic Cramér-Rao bound of the corresponding estimation problem is presented, illustrating the interest of geometry for performance analysis. These results are validated with simulations.
We consider the problem of random-design linear regression, in a distribution-free setting where no assumption is made on the distribution of the predictive/input variables. After surveying existing approaches and indicating some improvements, we explain why they fall short in our setting. We then identify the minimal assumption on the target/output under which guarantees are possible, and describe a nonlinear prediction procedure achieving the optimal error bound with high probability.