e-Science
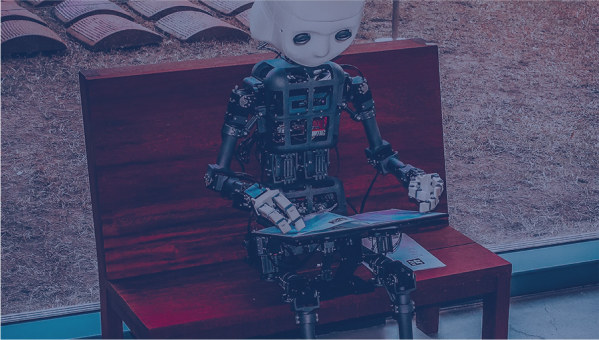
The revolution in experimental techniques has opened the door to very large datasets with a limited number of observations. A key challenge is to support this change by developing a culture of data expertise.
Scientific disciplines generate large amounts of data through experiments. A large part of a scientist's work consists in exploiting this data: storing it, representing it, extracting useful or important information from it, then modeling it, developing statistical models, making predictions, testing it and so on. As this data becomes increasingly complex, not all scientists are necessarily equipped to meet the challenges posed by this data, which is becoming more and more technical as time goes by.
The DATAIA Institute offers tools to make data analysis easier for scientists.
To create new collaborations and energize data analysis, one new approach is to support the creation of challenges to identify the best strategies for a given problem, and combine them to arrive at a more effective overall model. As part of the Center for Data Science, researchers and engineers from the Parietal team at the Inria Saclay - Île-de-France center, launched a challenge on autism in 2018 based on images of the brains of children, autistic or not. In this way, learning specialists are developing predictive models for diagnosing the disease. This challenge has advanced the prediction score and boosted the quality of autism diagnosis by 10%.Within the e-sciences framework, another type of approach involves establishing the causal structure of phenomena rather than prediction: this is a very active branch of learning.