« STARS » project
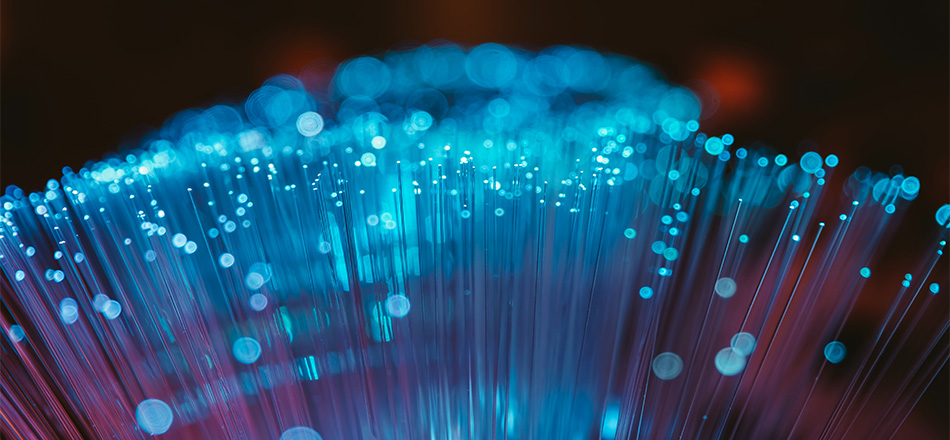
The aim of the project is to build innovative, expressive and flexible models for the computational analysis of the musical structure of audio signals for exploration and interaction with large audio databases, and for a better understanding of the ability of human beings to get an idea of how music is organized.
This project is highly interdisciplinary, bringing together efforts from different scientific fields (signal processing, statistical relational learning, deep learning, large-scale data analysis), but also knowledge from humanistic disciplines such as music analysis, musicology and jazz music studies. It benefits from the combination of the various fields of expertise of the different members involved in the team. In addition to developing innovative models for music processing, it addresses AI methodological issues and also contributes to interaction with related digital humanities disciplines.
A paper accepted in the ISMIR conference, the most important in the Music Information Retrieval MIR field: Morgan Buisson, Brian McFee, Slim Essid, Hélène-Camille Crayencour, Learning Multi-Level Representations for Hierarchical Music Structure Analysis, 2022
Project leaders
- Hélène-Camille Crayencour (L2S - CNRS) - Expert in relational statistical learning and music information retrieval;
- Slim Essid (LTCI, Télécom Paris, Institut Polytechnique de Paris) - Expert in structured prediction, representation learning and audio signal processing;
- Matthieu Kowalski (L2S - Paris-Saclay University) - Expert in structured sparse approximations and data decomposition methods.