« StreamOps » project
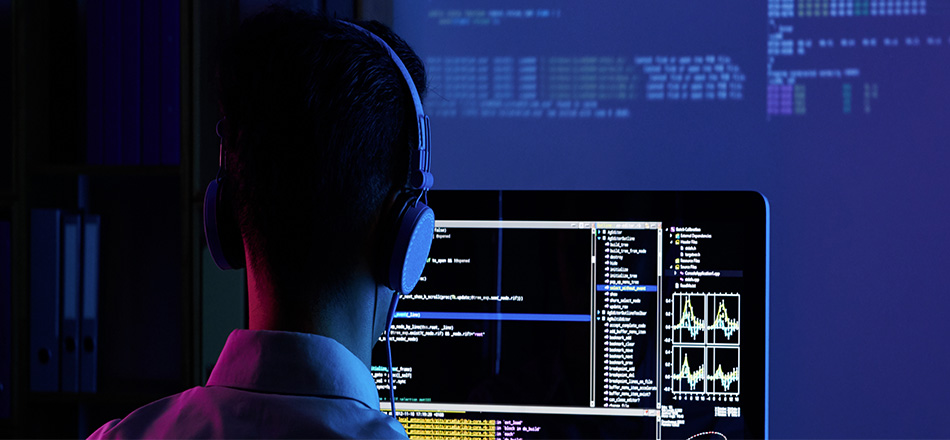
The scientific community is developing algorithms to manage data flows. Industry, on the other hand, is seeking to analyze the subject from a more applicative angle.
Karine Zeitouni, Yehia Taher (DAVID Laboratory, Versailles Saint-Quentin-en-Yvelines University) and Cédric Gouy-Pailler (Data Analysis and Systems Intelligence Laboratory, CEAList) have decided to combine their skills to offer the scientific community a simple new tool for developing powerful algorithms capable of handling data flow problems. This tool will be applied in particular in the medical field, in collaboration with Philippe Aegerter (Inserm UMR 1168) and Marc Fischler (Hôpital Foch, Université Versailles Saint-Quentin-en-Yvelines).
A generic yet cutting-edge streaming platform
In IT, extremely powerful tools in terms of data throughput and robustness are being developed. “With the StreamOps project, we want to position ourselves at the interface of algorithmic, business and software aspects, to offer all players a streaming platform that is generic but cutting-edge in terms of algorithms”, explains Cédric. Indeed, StreamOps' ambition is to simultaneously meet the following objectives:
-
Detection performance (responsiveness, accuracy), information compression performance, data confidentiality considerations;
-
Consideration of problems linked to real data (missing data, sensor problems);
-
Ease of integration of new algorithms;
-
Operational robustness (high data throughput, robustness to node failures).
Data flows from environmental and health sensors
Karine has been working with Yehia for several years on the Polluscope project (ANR) and with Philippe on ACE-ICSEN, an IRS project at the Université Paris-Saclay. They met Cédric at the Center for Data Science and decided to work together to raise the issue of data management, data analysis and Machine Learning on IoT data.
On the one hand, StreamOps will use a sample of Polluscope data collected by multi-sensor handheld devices as part of a participatory data collection process. The aim of Polluscope is to analyze, in all dimensions, all pollution data to characterize an individual's exposure to air pollution. The SteamOps project will contribute to the analysis and Machine Learning of these data streams. At the same time, Philippe and Marc are about to test a connected multi-signal physiological sensor (patch) for pre-operative and especially post-operative medical monitoring. The idea is to attach a multi-sensor patch to the patient's thorax, so as to monitor him or her remotely and continuously in the days following the operation, in order to anticipate the risk of complications and trigger the right alert thanks to an intelligent decision support system, without having to keep the patient in a specialized medical unit. The aim of StreamOps is to use these two types of data to create a generic application.
Se positionner à l'interface
Karine précise : « Nous allons développer de nouveaux algorithmes qui feront l’interface entre une communauté qui voit les données comme des séries temporelles et qui les analyse d’un point de vue historique, et une autre qui voit l’IoT comme un flux de données et analyse l’ensemble de ces données de manière dynamique, au fur et à mesure de leur enregistrement. » L’objectif de StreamOps est de développer des méthodes et des algorithmes pour considérer l’ordre temporel dans les flux de données. Cédric travaille régulièrement avec des industriels également intéressés par la possibilité d’avoir des outils automatiques pour traiter les données qui viennent en flux. « Il ne s’agit pas de proposer une plate-forme de plus, explique Karine, mais une plate-forme intégratrice. » L’équipe StreamOps compte notamment collaborer avec Albert Bifet, Telecom ParisTech, qui a développé la plate-forme MOA (Massive Online Analysis) afin de créer une plateforme compatible et arriver à établir des synergies.
Positioning ourselves at the interface
Karine explains: “We're going to develop new algorithms that will interface between a community that sees data as time series and analyzes them from a historical point of view, and another that sees the IoT as a data stream and analyzes all this data dynamically, as it is recorded.” The aim of StreamOps is to develop methods and algorithms for considering the temporal order of data flows. Cédric works regularly with manufacturers who are also interested in the possibility of having automatic tools for processing data that comes in streams. "It's not a question of offering yet another platform, but an integrating platform", explains Karine. In particular, the StreamOps team intends to collaborate with Albert Bifet of Telecom ParisTech, who developed the MOA (Massive Online Analysis) platform, to create a compatible platform and establish synergies.
Contacts : Karine Zeitouni | Cédric Gouy-Pailler | Yehia Taher