« UltraBioLearn » project
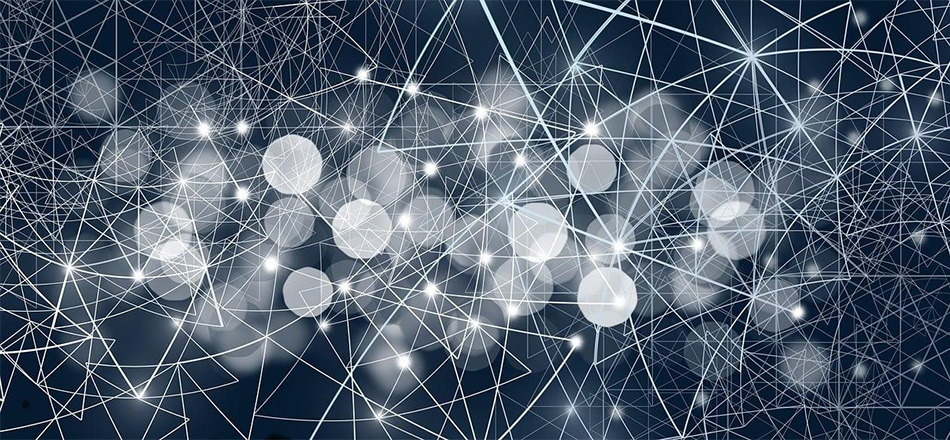
Machine learning for medical applications, and supervised deep learning in particular, imposes constraints that are difficult to verify: results must be interpretable, of good quality, reproducible and auditable.
We also know that performance depends on the quality of the learning base and annotations. At the same time, learning from medical data requires respect for patient privacy. In Europe, access to medical data is restricted. The combination of these factors makes the use of deep learning for imaging difficult in practice.
The aim of this research project is to find innovative solutions to these questions, while adding an important application context: the use of these techniques in the context of immunotherapy in oncology.
The aim of this project is to propose an approach based on the very structure of the architectures employed, enabling a priori regularization of the trained networks so as to enable faster, safer and more efficient convergence of the proposed antagonistic generative networks. In terms of applications, the project proposes to implement these networks in the classification of tumor lesions in ultrasound and CT scans, in order to obtain a rapid and objective assessment of patient response to immunotherapeutic treatment in cancerology.
The project is therefore ambitious, because in this field, data and annotations are difficult to obtain. A CVN-LR4M collaboration will make it possible to produce a sufficient quantity of these data to be able to initiate training and demonstrate that the approach is feasible, through the collaboration of the two teams within the Institut Gustave-Roussy. At application level, the aim will be to demonstrate the good properties of the networks obtained, by applying them to the problem of obtaining (1) segmentations of lesions in ultrasound and CT; (2) characterizations of these lesions in the context of immunotherapy. A conditional extension of the proposed deep learning architecture could enable this type of application.
Contacts : Hugues Talbot | Fragkiskos Malliaros | Nathalie Lassau