« Le Séminaire Palaisien » | Arnak Dalalyan et Frédéric Pascal sur l'apprentissage automatique et la statistique
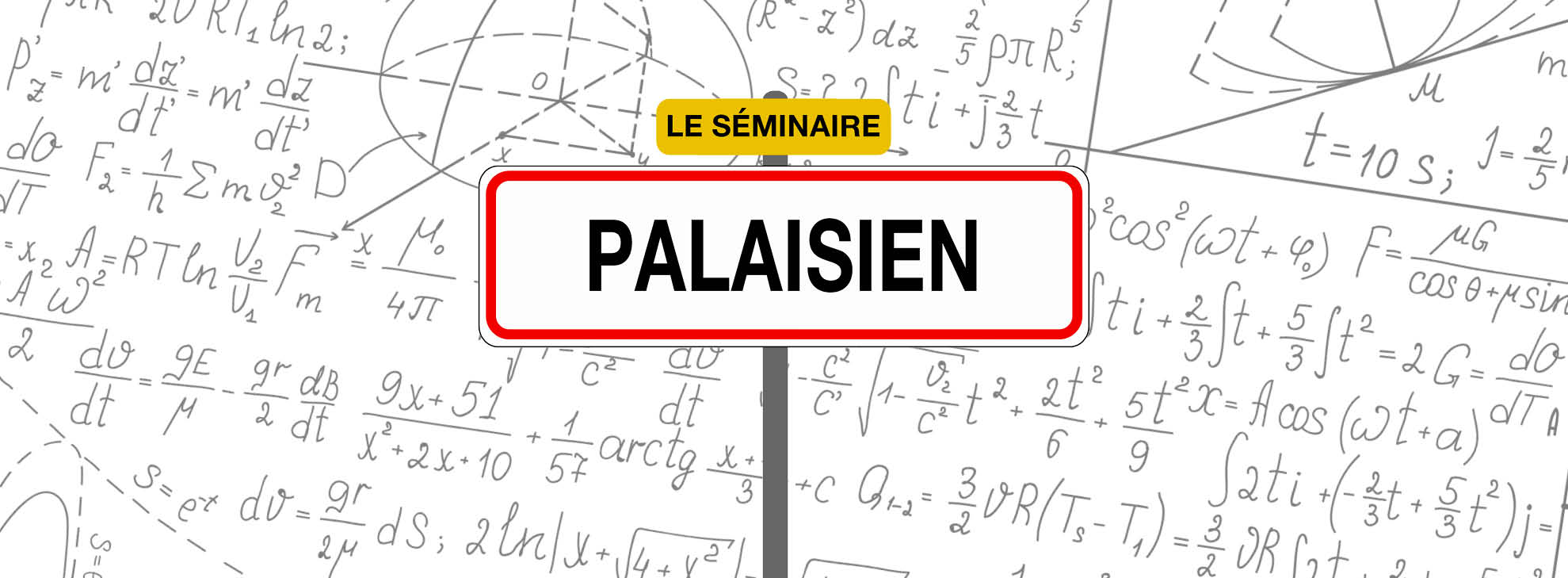
Chaque session du séminaire est divisée en deux présentations scientifiques de 40 minutes chacune : 30 minutes d’exposé et 10 minutes de questions.
Arnak Dalalyan (ENSAE) et Frédéric Pascal (CentraleSupélec) animeront la session de février 2022.
The goal of this talk is to show that a single robust estimator of the mean of a multivariate Gaussian distribution can enjoy five desirable properties. First, it is computationally tractable in the sense that it can be computed in a time which is at most polynomial in dimension, sample size and the logarithm of the inverse of the contamination rate. Second, it is equivariant by scaling, translations and orthogonal transformations. Third, it has a nearly-minimax-rate-breakdown point approximately equal to 0.28. Fourth, it is minimax rate optimal when data consist of independent observations corrupted by adversarially chosen outliers. Fifth, it is asymptotically optimal when the rate of contamination tends to zero. The estimator is obtained by an iterative reweighting approach. Each sample point is assigned a weight that is iteratively updated using a convex optimization problem. We also establish a dimension-free non-asymptotic risk bound for the expected error of the proposed estimator. It is the first of this kind results in the literature and involves only the effective rank of the covariance matrix.
(Joint work with Arshak Minasyan)
Though very popular, it is well known that the EM algorithm suffers from non-Gaussian distribution shapes, outliers and high-dimensionality. In this talk, we introduce a new robust clustering algorithm that can efficiently deal with noise and outliers in diverse data sets. As an EM-like algorithm, it is based on both estimations of clusters centers and covariances. In addition, using a semi-parametric paradigm, the method estimates an unknown scale parameter per datapoint. This allows the algorithm to accommodate for heavier tails distributions and outliers without significantly loosing efficiency in various classical scenarios. Then, we show that the proposed algorithm outperforms other classical unsupervised methods of the literature such as k-means, the EM algorithm and its recent modications or spectral clustering when applied to real data sets as MNIST, NORB and 20newsgroups. An application to Radar (SAR) image segmentation is proposed, highlighting the interest of the proposed approach.