« Le Séminaire Palaisien » | David Degras & Jordan Serres
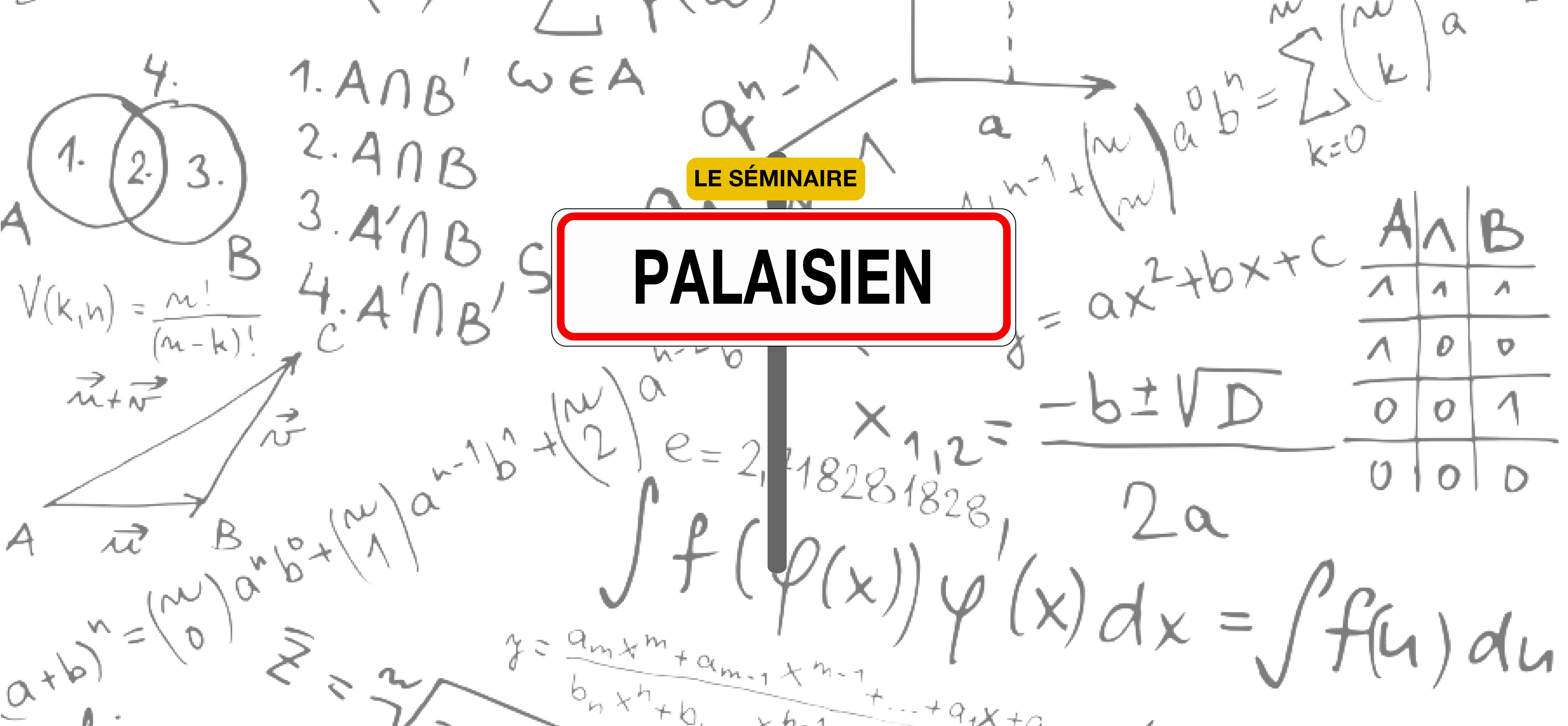
Chaque session du séminaire est divisée en deux présentations scientifiques de 40 minutes chacune : 30 minutes d’exposé et 10 minutes de questions.
David Degras et Jordan Serres animeront la session d'octobre 2023 !
Inscriptions gratuites mais obligatoires, dans la limite des places disponibles. Un buffet sera servi à l'issue du séminaire.
Résumé : This talk introduces the sparse group fused lasso (SGFL), a statistical framework for segmenting sparse regression models of multivariate time series. To compute solutions of the SGFL, a nonsmooth and nonseparable convex program, we develop a hybrid optimization method that is fast, requires no internal tuning, and is guaranteed to converge to a global minimizer. In numerical experiments, the proposed algorithm compares favorably to the state of the art with respect to computation time and numerical accuracy; benefits are particularly substantial in high dimension. Statistical performance is satisfactory in recovering nonzero regression coefficients and excellent in change point detection. Applications to air quality and neuroimaging data will be presented. The hybrid algorithm is implemented in an R package available here.
Résumé : Barycenters in non-Euclidean geometries are the most natural extension of linear averaging. They are widely used in shape statistics, optimal transport and matrix analysis. In this talk, I will present their asymptotic properties, i.e. law of large numbers. I will also present some more recent results about their non-asymptotic concentration rate.