« Le Séminaire Palaisien » | Gabriel Peyré & Tom Sanders
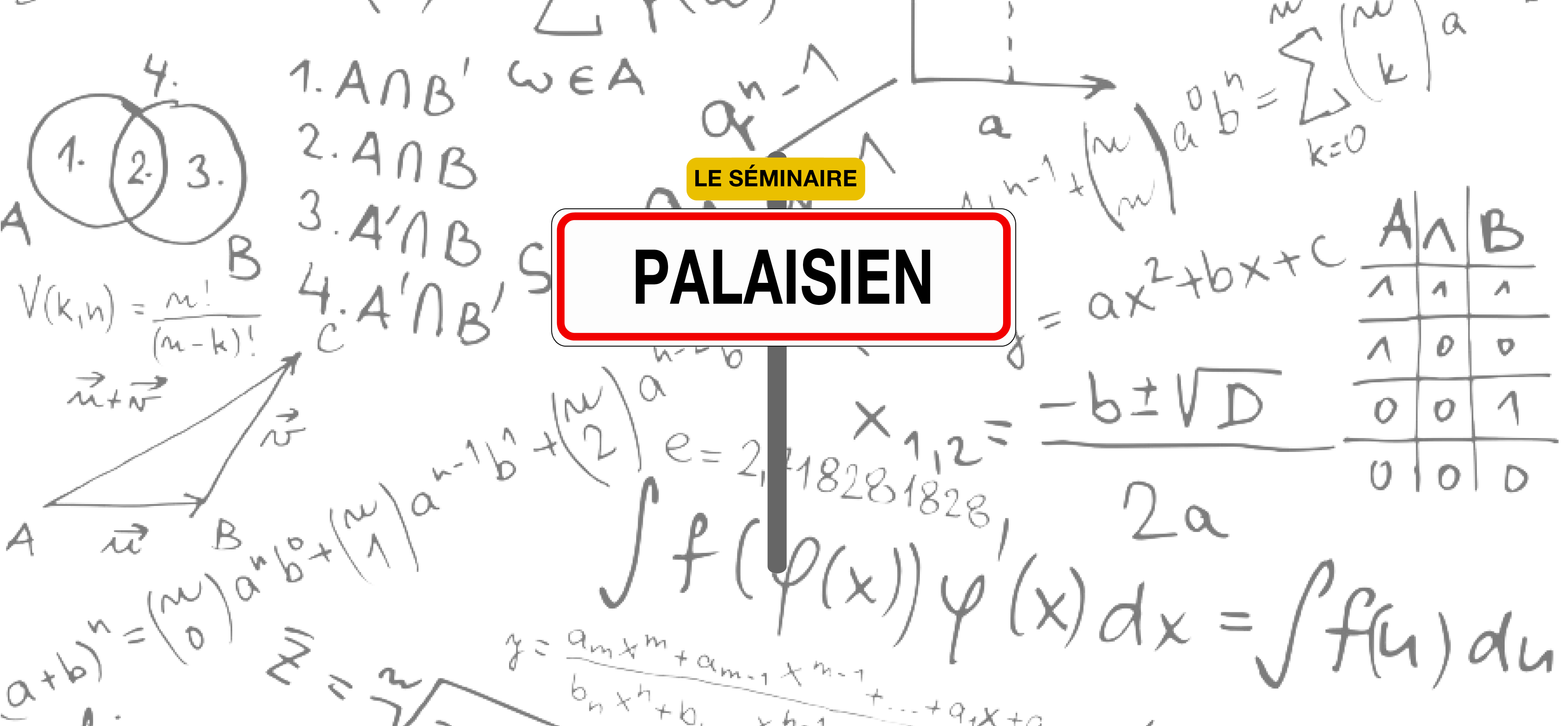
Chaque session du séminaire est divisée en deux présentations scientifiques de 40 minutes chacune : 30 minutes d’exposé et 10 minutes de questions. Gabriel Peyré & Tom Sanders animeront la session de mars 2025 !
Inscriptions gratuites mais obligatoires, dans la limite des places disponibles. Un buffet sera servi à l'issue du séminaire.
Résumé
Temporal point processes (TPP) are a natural tool for modeling event-based data. Among all TPP models, Hawkes processes have proven to be the most widely used, mainly due to their adequate modeling for various applications, particularly when considering exponential or non-parametric kernels. Although non-parametric kernels are an option, such models require large datasets. While exponential kernels are more data efficient and relevant for specific applications where events immediately trigger more events, they are ill-suited for applications where latencies need to be estimated, such as in neuroscience. In this talk, we will present an efficient solution to Hawkes inference using general parametric kernels with finite support. The developed solution consists of a fast L2 gradient-based solver leveraging a discretized version of the events. After theoretically supporting the use of discretization, the statistical and computational efficiency of the novel approach is demonstrated through various numerical experiments. Finally, the method’s effectiveness is evaluated by modeling the occurrence of stimuli-induced patterns from brain signals (M/EEG) and ECG.
Résumé
Invisible watermarking robustly embeds binary messages within data. This presentation begins with an introduction to watermarking fundamentals and its applications in copyright protection and Generative AI content detection. The focus will then be on image watermarking, highlighting Watermark Anything, which approaches watermarking as a segmentation task, enabling tamper detection and multiple message hiding, as well as improved robustness. Finally, the presentation explores text watermarking and sheddes light on its "radioactive" properties, meaning that watermarked text propagates through training, which can help detect model distillation and test-set contamination.