« Le Séminaire Palaisien » | Zaccharie Ramzi et Emilie Chouzenoux sur l'apprentissage automatique et la statistique
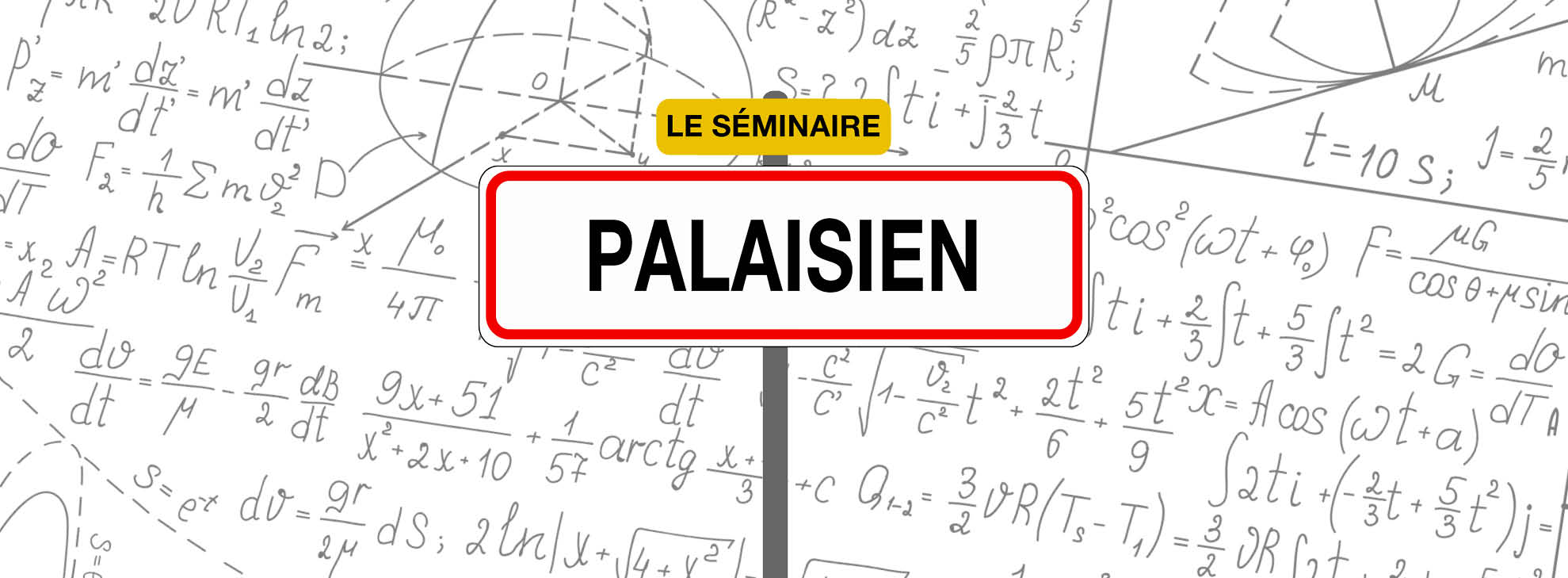
Chaque session du séminaire est divisée en deux présentations scientifiques de 40 minutes chacune : 30 minutes d’exposé et 10 minutes de questions.
Emilie Chouzenoux (Inria) et Zaccharie Ramzi (CEA) animeront la session de février 2021.
The proximal gradient algorithm is a popular iterative algorithm to deal with penalized least-squares minimization problems. Its simplicity and versatility allow one to embed nonsmooth penalties efficiently. In the context of inverse problems arising in signal and image processing, a major concern lies in the computational burden when implementing minimization algorithms. For instance, in tomographic image reconstruction, a bottleneck is the cost for applying the forward linear operator and its adjoint. Consequently, it often happens that these operators are approximated numerically, so that the adjoint property is no longer fulfilled.
In this talk, we focus on the proximal gradient algorithm stability properties when such an adjoint mismatch arises. By making use of tools from convex analysis and fixed point theory, we establish conditions under which the algorithm can still converge to a fixed point. We provide bounds on the error between this point and the solution to the minimization problem. We illustrate the applicability of our theoretical results through numerical examples in the context of computed tomography.
This is joint work with M. Savanier, J.C. Pesquet, C. Riddell and Y. Trousset
References :
[1] E. Chouzenoux, J.C. Pesquet, C. Riddell, M. Savanier and Y. Trousset. Convergence of Proximal Gradient Algorithm in the Presence of Adjoint Mismatch. To appear in Inverse Problems, 2020. http://www.optimization-online.org/DB_HTML/2020/10/8055.html
[2] M. Savanier, E. Chouzenoux, J.C. Pesquet, C. Riddell and Y. Trousset. Proximal Gradient Algorithm in the Presence of Adjoint Mismatch. In Proceedings of the 28th European Signal Processing Conference (EUSIPCO 2020), January 18-22 2021
In classical Magnetic Resonance Imaging reconstruction, slow iterative non-linear algorithms using manually crafted priors are applied to obtain the anatomical image from under-sampled Fourier measurements. In addition they have to deal with an incomplete knowledge of the exact measurement operator.
Deep Learning methods, and in particular, unrolled networks, have allowed to alleviate those issues. In this talk we will see how Deep Learning enables us to:
- Learn an optimal optimization scheme,
- Learn a prior from the data
- Learn how to refine our knowledge of the measurements operator.
We show the results of this approach on the fastMRI 2020 brain reconstruction challenge where we secured the 2nd spot in both the 4x and 8x acceleration tracks.