Séminaire DATAIA | David Degras "Joint Tensor Decomposition: Methods and Models"
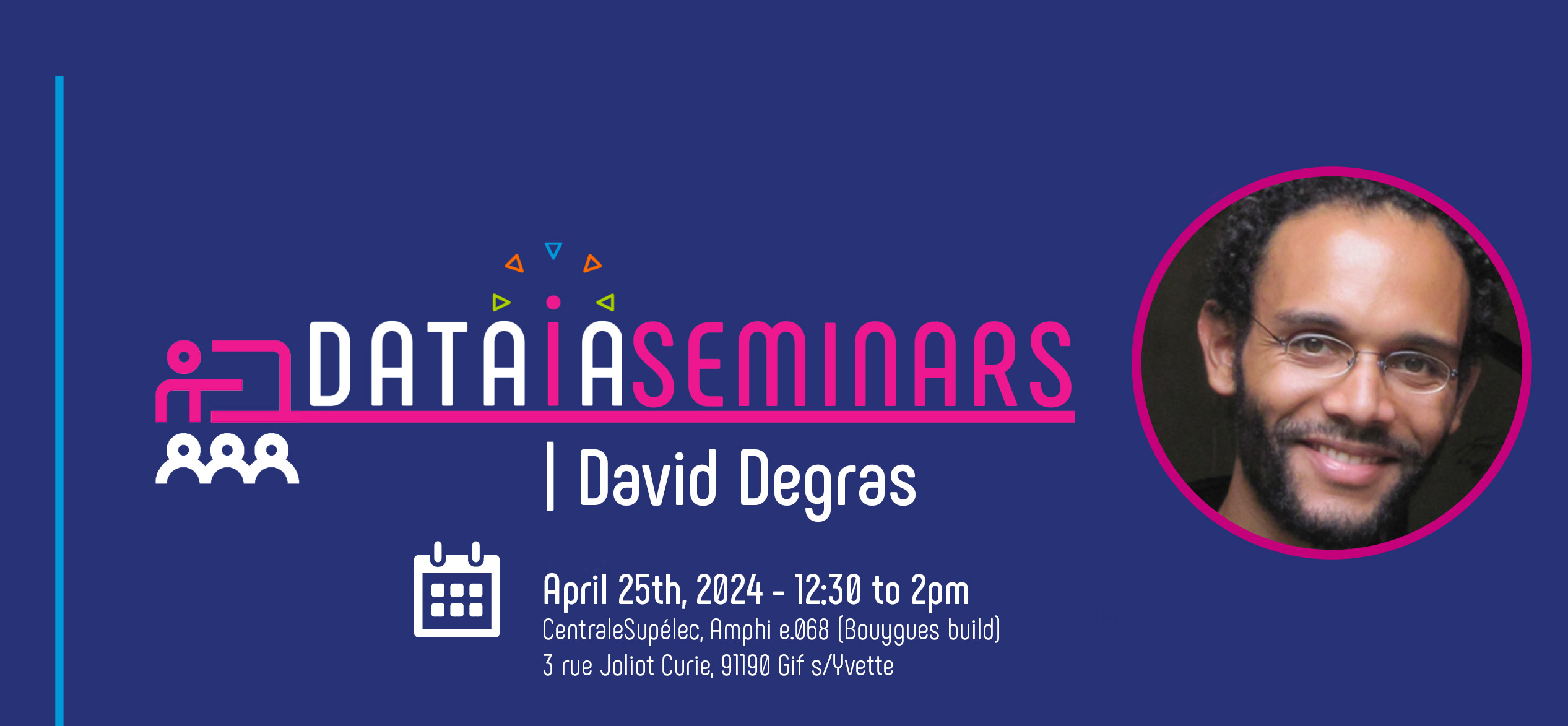
Titre
Joint Tensor Decomposition: Methods and Models
Résumé
In science and industry, data often arise as tensors, or multidimensional arrays, collected along various dimensions such as time, space, or frequency. Examples include video sequences in computer vision, 2D+ images in engineering and biomedical research, audio signals, and text embeddings in natural language processing. Preserving tensor structure in analysis can provide significant statistical and computational advantages over routine vectorization methods. Tensors retain the inherent multidimensional relationships within data, leading to more accurate and interpretable representations of complex phenomena. Additionally, tensor operations enable efficient manipulation of high-dimensional data, resulting in substantial savings in computation time and memory usage.
However, the mathematical theory of tensors remains somewhat elusive and is still under active development. While the maximum rank of a matrix of given dimensions is well understood, determining the maximum rank of a tensor is an open problem. Similarly, while the rank of a matrix can be easily determined using established algorithms like QR or SVD, finding the rank of a tensor is generally NP-hard. There is ample room for theoretical advances in tensor algebra and geometry, as well as in tensor-based optimization and statistics.
In this talk, I will delve into the problem of joint tensor decomposition, which involves identifying common variations among multiple tensor datasets collected on the same objects or persons, also known as data fusion or integration in the literature. After reviewing standard tensor decompositions, I will focus on tensorial extensions of partial least squares (PLS) and canonical correlation analysis (CCA), presenting novel algorithms based on block coordinate ascent or Riemannian gradient optimization that can jointly decompose multiple tensor datasets of arbitrary orders. I will provide numerical convergence results and statistical guarantees within the context of factor models. Moreover, I will discuss algorithm initialization, higher-order factor components, and statistical inference methods such as bootstrap and permutation techniques. If time permits, I will showcase numerical evidence of the method’s performance and outline potential applications in the multimodal integration of neuroimaging data.
Affiliation
University of Massachusetts (Boston, USA)
Professeur Associé - Département Mathématiques
Invited Professor (2023-24)
Inria Saclay & CEA Saclay - MIND team
Biographie
David Degras a obtenu son doctorat en statistiques à l'Université Paris 6, France, en 2008. Il a été chercheur postdoctoral à l'Institut de statistique et de sciences mathématiques appliquées (SAMSI) en 2010-2011 et a été professeur adjoint au département de sciences mathématiques de l'université DePaul de 2011 à 2016. Il est actuellement professeur associé au département de mathématiques de l'université du Massachusetts à Boston. Ses recherches portent sur l'apprentissage statistique, l'informatique statistique, l'analyse des données fonctionnelles, l'optimisation convexe et combinatoire et la neuro-imagerie.
- Le séminaire aura lieu le jeudi 25 avril 2024, de 12h30 à 14h à CentraleSupélec, amphithéâtre e.068 (bâtiment Bouygues) à Gif s/Yvette.
- Ce séminaire sera également retransmis en visioconférence : https://teams.microsoft.com/l/meetup-join/19%3ameeting_M2ZkMDVlNTgtNzAwNS00YTlkLWI2NmMtMjhmNGZiODE3MTdj%40thread.v2/0?context=%7b%22Tid%22%3a%2261f3e3b8-9b52-433a-a4eb-c67334ce54d5%22%2c%22Oid%22%3a%2240a0f890-4bbf-4d69-92b9-e53f47d70bf7%22%7d
Ne ratez pas l'annonce d'un nouveau séminaire DATAIA !
Inscrivez-vous à la liste de diffusion de nos séminaires en cliquant ici.