Séminaire DATAIA | José Dolz - Towards robust and efficient adaptation of vision-language foundation models
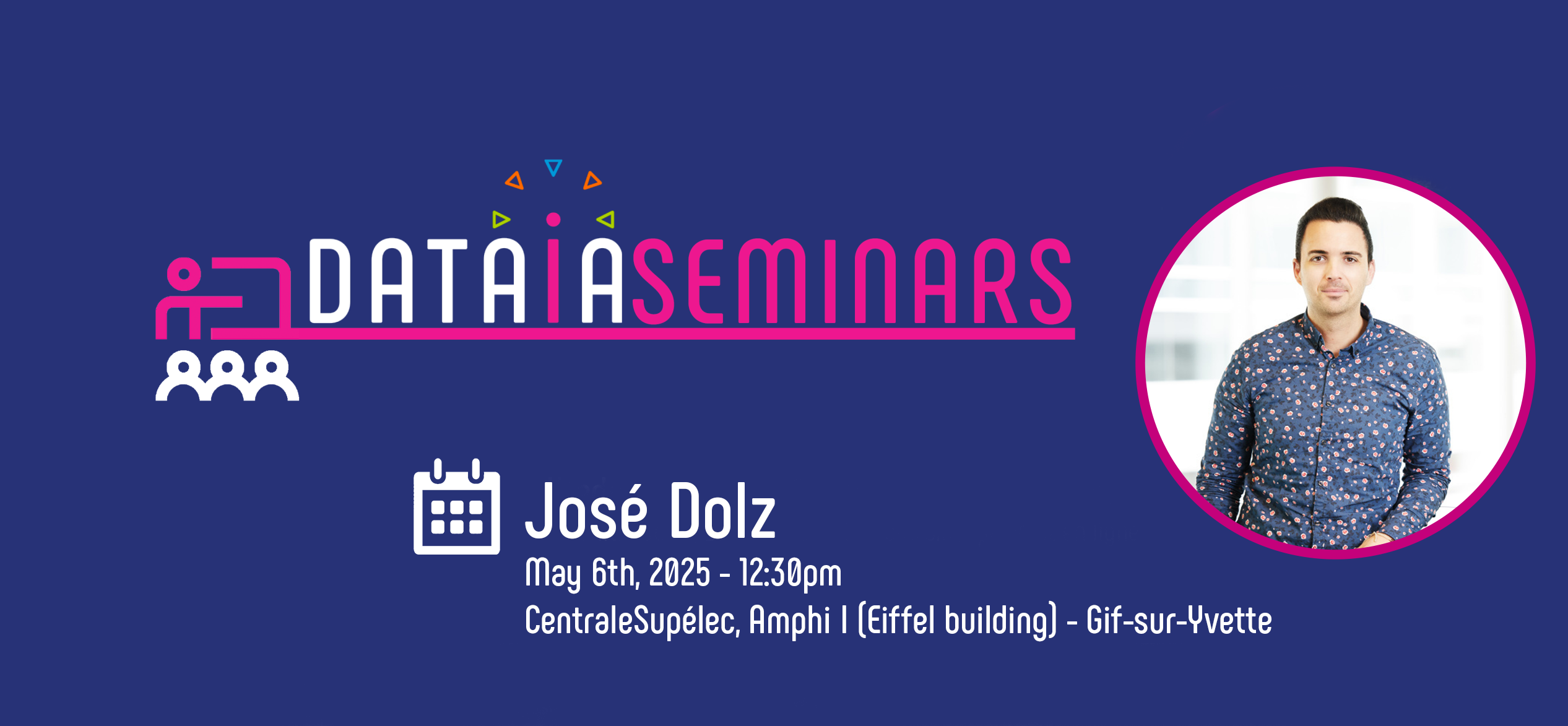
Title
Towards robust and efficient adaptation of vision-language foundation models
Abstract
Deep learning (DL) has achieved remarkable performance in a wide span of visual recognition problems in strategic areas for our society, such as healthcare, video-surveillance, or autonomous driving. In particular, vision-language models (VLMs) trained at large-scale have emerged recently as a new learning paradigm, showcasing unprecedented zero-shot and transfer capabilities. Nevertheless, they present important drawbacks that limit their deployment in real-world scenarios. First, despite the astonishing performance achieved by VLMs, these do not generalize well to unseen scenarios, such as novel classes or distributions presenting a domain shift, typically requiring large labeled training datasets for each novel task. However, obtaining such annotations could be a cumbersome process in several domains, such as healthcare, which may also require user-expertise and suffers from inter/intra-rater variability. A common practice to adapt VLMs to novel tasks involves fine-tuning a pre-trained model (such as CLIP) with annotated samples of the target task. Even if this technique can make VLM's use more general, it increases the computational burden, making it suboptimal in scenarios with limited access to data and annotations. Moreover, recent evidence exposes that adapted VLMs suffer from poor calibration, i.e. the confidence scores of the predictions do not reflect the real world probabilities of those predictions being true. Thus, these models tend to produce overconfident estimates, even in situations of high uncertainty, leading to poorly calibrated and unreliable models. This is further magnified if the model is adapted under a low labeled data regime, a popular learning paradigm to alleviate the need of large labeled training datasets to adapt VLMs such as CLIP. Thus, in this talk we will discuss different approaches to efficiently adapt VLMs to novel tasks presenting either a domain or a label shift, and how to better model the uncertainty estimations.
Biography
José Dolz is an Associate Professor in the Department of Software and IT Engineering at the ETS Montreal. Prior to be appointed Professor, he was a post-doctoral fellow at the same institution. He obtained his B.Sc and M.Sc in the Polytechnic University of Valencia, Spain, and his Ph.D. at the University of Lille 2, France, in 2016. José was recipient of a Marie-Curie FP7 Fellowship (2013-2016) to pursue his doctoral studies. His current research focuses on deep learning, medical imaging, optimization and learning strategies with limited supervision. Up to date, he has (co-)authored over 80 fully peer-reviewed papers, many of which published in the top venues in medical imaging (MICCAI/IPMI/MedIA/TMI/NeuroImage), computer vision (CVPR, ICCV, ECCV) and machine learning (ICML, NeurIPS). Furthermore, he has given 5 tutorials on learning with limited supervision at MICCAI (2019-2022) and ICPR(2022), and one in foundation models (MICCAI 2024) participated in the organization of three summer schools in Deep Learning for Medical Imaging and recognized several times as Outstanding Reviewer (MICCAI'20, ECCV'20, CVPR'21, CVPR'22, NeurIPS'22, ICCV'23).
- The seminar will take place on Tuesday, May 6, 2025, from 12.30 pm to 2 pm at CentraleSupélec, Amphi I (Eiffel building) in Gif-sur-Yvette ;
- A coffee break will be served afterwards.