« Seminar Le Palaisien » | Alexandre Perez and Marco Cuturi
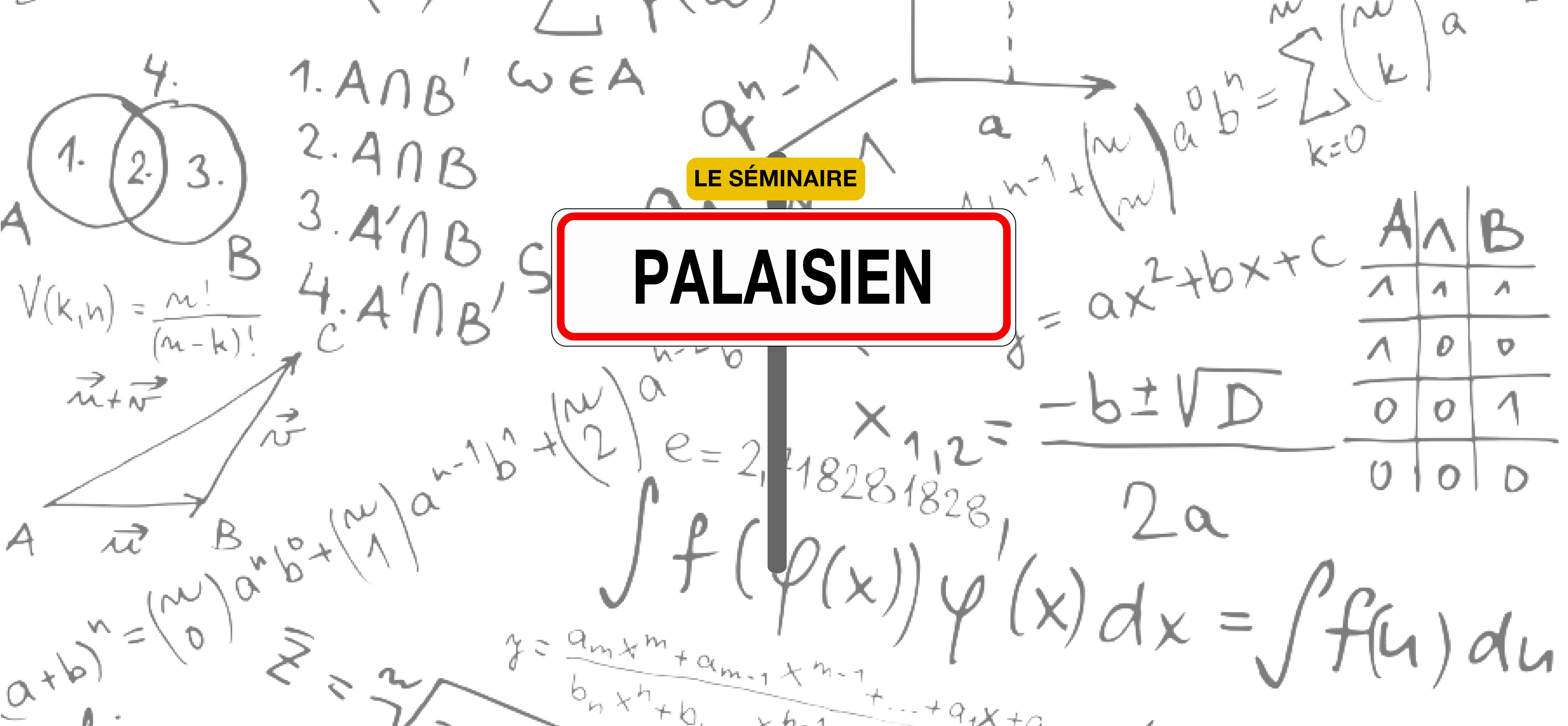
Each seminar session is divided into two scientific presentations of 40 minutes each: 30 minutes of presentation and 10 minutes of questions.
Alexandre Perez-Lebel and Marco Cuturi will lead the December 2022 session.
Registration is free but mandatory, subject to availability. A sandwich basket is offered.
Abstract: Good decision making requires machine-learning models to provide trustworthy confidence scores. To this end, recent work has focused on miscalibration, i.e, the over or under confidence of model scores. Yet, contrary to widespread belief, calibration is not enough: even a classifier with the best possible accuracy and perfect calibration can have confidence scores far from the true posterior probabilities. This is due to the grouping loss, created by samples with the same confidence scores but different true posterior probabilities. Proper scoring rule theory shows that given the calibration loss, the missing piece to characterize individual errors is the grouping loss. While there are many estimators of the calibration loss, none exists for the grouping loss in standard settings. Here, we propose an estimator to approximate the grouping loss. We use it to study modern neural network architectures in vision and NLP. We find that the grouping loss varies markedly across architectures, and that it is a key model-comparison factor across the most accurate, calibrated, models. We also show that distribution shifts lead to high grouping loss.
Abstract: I will present in this talk a series of efforts targeted at increasing the scalability and applicability of OT computations. I will present efforts on two fronts: In the first part, I will discuss speeding up the discrete resolution of the Kantorovich problem, using either the Sinkhorn approach, and, in that case, focusing on efficient heuristics to initialize Sinkhorn potentials, or, alternatively, by parameterizing OT couplings as a product of low-rank non-negative matrices. In the second part I will explain how a parameterization, in the 2-Wasserstein setting, of dual potentials as input-convex neural networks has opened several research avenues, and demonstrate this by illustrating a recent application to a multitask, joint estimation of several Monge maps linked by a common set of parameters.