« Seminar Le Palaisien » | Rémi Flamary & François Lanusse
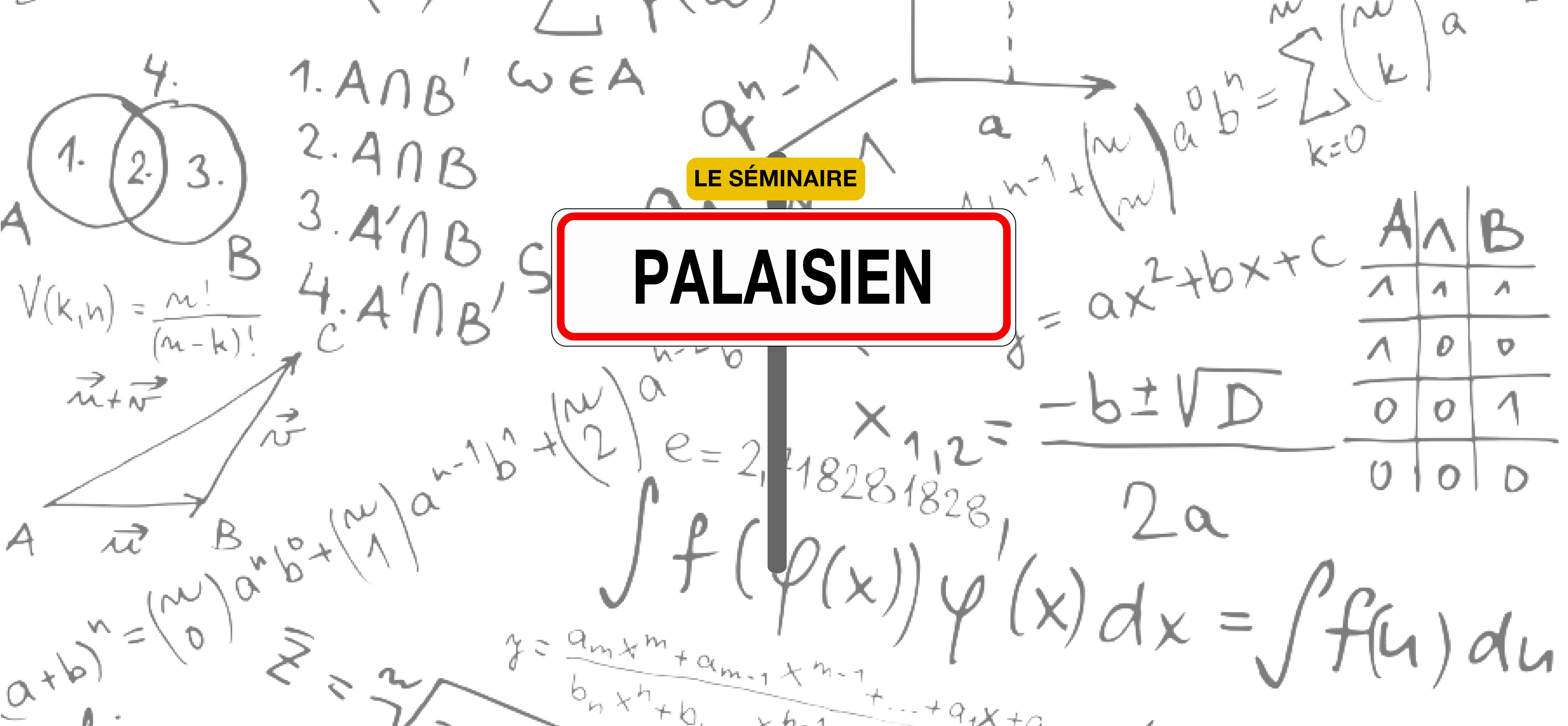
Each seminar session is divided into two scientific presentations of 40 minutes each: 30 minutes of presentation and 10 minutes of questions.
Rémi Flamary and François Lanusse will moderate the May 2023 session.
Registration is free but mandatory, subject to availability. A buffet will be served at the end of the seminar.
Abstract: In recent years the Optimal Transport (OT) based Gromov-Wasserstein (GW) divergence has been investigated as a similarity measure between structured data expressed as distributions typically lying in different metric spaces, such as graphs with arbitrary sizes. In this talk, we will address the optimization problem inherent in the computation of GW and some of its recent extensions, namely the Entropic and the Fused GW divergences. Next we will illustrate how these OT problems can be used to model graph data in learning scenarios such as graph compression, clustering and classification. Finally we will present a trecent application of GW distance as a novel pooling layer in Graph Neural Networks.
Abstract: Modeling probabilities over 3D orientations is an important area of directional statistics which finds a wide range of applications from computer vision and robotics to physics. In this talk I will present a new approach for modeling conditional distributions on SO(3) (the Lie group of 3D rotations) based on extending Euclidean Score-Based Generative models (e.g. Song et al. 2021) to the SO(3) manifold. From a methodological point of view, our implementation relies on specific properties of heat diffusion on SO(3) that allow for closed-form solutions of the diffusion Stochastic Differential Equation (SDE) on that manifold, as well as making use of geometric Ordinary Differential Equation (ODE) solvers to efficiently sample and evaluate the log probability of the model.
He will then illustrate a concrete application of these conditional generative models to emulate some of the complex physics present in cosmological hydrodynamics simulations, but too costly to simulate on large simulation volumes. In particular, he will show how the SO(3) model can correctly capture and reproduce the complex dependence of the 3D orientations of galaxies with their environment, an effect that is very important to model correctly for analyzing cosmological observations in practice.