CDS@DATAIA Challenges
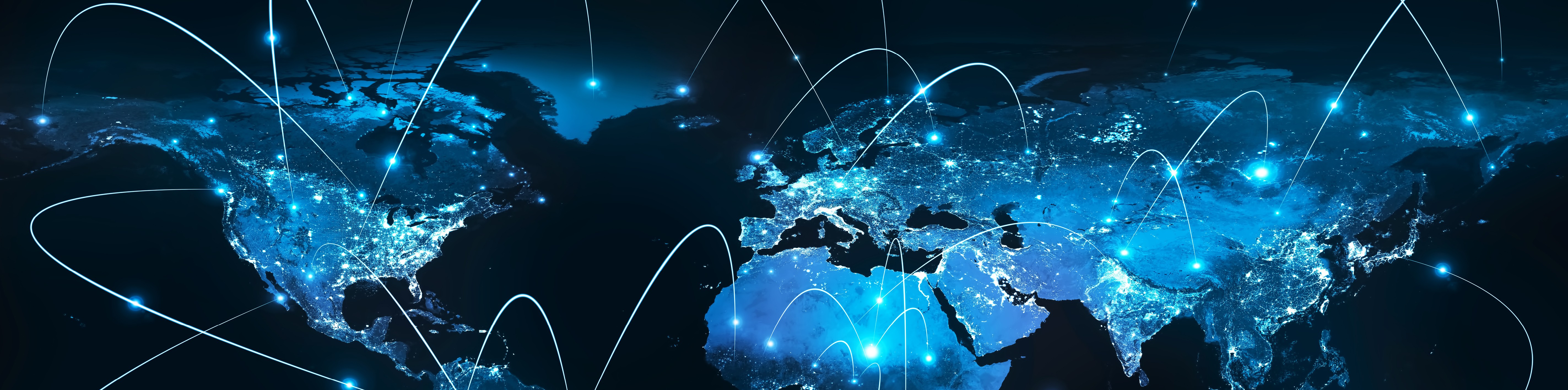
Implemented by the Paris-Saclay Center for Data Science (CDS) and co-organized with the DATAIA Institute, these various machine learning challenges are designed for students of the University of Paris-Saclay, to facilitate and help research. The main objective of these "data challenge competitions" is to respond more precisely to research problems, by bringing together scientists and students.
This approach is meant to be pedagogical and relies on the specificities of the RAMP software, a platform dedicated to collaborative prototyping (using the Python language), which allows the submission of the code and not only of the prediction vector, as it is usually done in machine learning challenges (Kaggle for example). The challenge organizers can thus retrieve a whole set of code prototypes ranked with the score of a chosen metric.
What is RAMP?
The Rapid Analytics and Model Prototyping (RAMP) software was initially developed by the Paris-Saclay Data Science Center (CDS) to connect data science to other scientific domains. The initial goal was to enable collaborative prototyping of machine learning workflows, in order to solve the data analysis segment of scientific problems. This tool is based on the willingness of participants to submit their own code and not just results. Each solution is evaluated by a remote server or in a cloud, and then published to maximize the plurality of solutions. The CDS team also launched RAMP.studio to put RAMP into action and host its own challenges. About 20 scientific challenges have emerged. CDS is now integrated into the DATAIA Institute.
It is in this dynamic that the RAMP platform, a prototyping system using open source, accessible to all, was born. This platform has been used for data challenges aimed at solving predictive problems, in scientific fields ranging from medicine, biology, neuroscience to astrophysics. These challenges usually result in a model with a significant improvement in prediction compared to the baseline.
Depending on the players involved, the challenges can take different forms (lasting on average 2 months): datacamp, event, open challenge, etc.
![]() |
This challenge, organized in August 2021, was carried out with the support of DATAIA Institute, in collaboration with the Institut de Radioprotection et de Sûreté Nucléaire (IRSN).
This challenge gathered 98 participants and 976 submissions.
Benjamin Dechenaux, Jean-Baptiste Clavel, Cécilia Damon (IRSN), François Caud, Alexandre Gramfort (DATAIA, Univ. Paris-Saclay)
The material contained in a nuclear reactor undergoes an irradiation which causes successive cascades of nuclear reactions, modifying its atomic composition. The knowledge of this composition evolving in time is an important parameter used to model the behavior of a nuclear reactor. But it is also a crucial element for safety studies related to its operation and a key element for the mitigation of a severe accident. Knowing the composition of a reactor at a given moment allows a rapid assessment of which radioactive isotopes may be released into the environment.
Modeling the evolution of the atomic composition of irradiated materials over time is usually done using time-consuming Monte Carlo simulations of the system under study. Although accurate, this computational scheme has proven to be inadequate in crisis (i.e. accidental) situations, where faster computational schemes must be developed.
This project aims at building a substitution model by machine learning able to predict the evolution of the nuclear inventory of a typical reactor of the French fleet.
![]() |
![]() |
This challenge was realized with the support of DATAIA Institute, in collaboration with INRIA, CNRS, INSERM and INRAE.
This challenge gathered 82 participants and 409 soumissions.
Frédérique Clément (INRIA), Raphäel Corre (CNRS), Céline Guigon (INSERM), François Caud, Benjamin Habert, Alexandre Gramfort (DATAIA, Univ. Paris-Saclay)
The challenge is to automatically detect and classify ovarian follicles on histological sections of mammalian ovaries.
The ovary is a unique example of a dynamic endocrine organ, undergoing permanent remodeling in adulthood. Ovarian function is supported by spheroid, multilayered, multiphasic structures, the ovarian follicles, which house the oocyte (female germ cell) and secrete a variety of hormones and growth factors. The ovary has a pool of follicles established early in life, which is gradually depleted by follicle development or death. Understanding the population dynamics of ovarian follicles is essential to characterize the reproductive physiological status of females from birth (or even prenatal life) to reproductive senescence.
Accurate estimation of the number of ovarian follicles at different stages of development is of paramount importance in the field of reproductive biology, for basic research, pharmacological and toxicological studies, as well as for clinical fertility management. Associated societal challenges include physiological ovarian aging (age-related fertility decline, menopause), pathological aging (premature ovarian failure), and toxicant-induced aging (endocrine disruptors, anticancer treatments).
In vivo, only the terminal stages of the follicles, i.e. the tip of the iceberg, can be monitored by ultrasound. To detect all follicles, invasive approaches, based on histology, are necessary. Ovaries are fixed, serially cut and stained with appropriate dyes and then manually analyzed by light microscopy. Such counting is a complex, tedious, operator-dependent and, above all, time-consuming procedure. To save time, only a few slices from an entire ovary are examined, which adds to the experimental noise and further degrades the reliability of the measurements.
Experimenters have high expectations for improving the classical counting procedure, and deep learning-based approaches to follicular counting could provide a significant advance in the field of reproductive biology.
Here we will distinguish 4 categories of follicles, from smallest to largest:
- Primordial;
- Primary ;
- Secondary ;
- Tertiary.
One of the difficulties is that there is a great disparity in size between all follicles. Another difficulty is that most of the pre-trained classification models are trained on everyday objects and not on biological tissues.
This challenge was realized with the support of DATAIA Institute, in collaboration with CEA NeuroSpin.
This challenge gathered 31 participants and 334 soumissions.
Edouard Duchesnay, Antoine Grigis (Université Paris-Saclay, CEA, NeuroSpin), François Caud, Alexandre Gramfort (Université Paris-Saclay, Institut DATAIA)
Introduction
Predicting age from brain gray matter (regression). Aging is associated with gray matter (GM) atrophy. Each year, an adult loses 0.1% of GM. We will attempt to learn a predictor of chronological age (true age) using brain MG measurements on a population of healthy control participants.
Such a predictor provides the expected brain age of a subject. A deviation from this expected brain age indicates an acceleration or slowing of the aging process that may be associated with a pathological neurobiological process or a protective factor for aging.
This challenge was realized with the support of DATAIA Institute, in collaboration with CEA NeuroSpin.
Edouard Duchesnay, Antoine Grigis (Université Paris-Saclay, CEA, NeuroSpin), François Caud, Alexandre Gramfort (Université Paris-Saclay, Institut DATAIA)
Introduction
The brainage_deep challenge is an extension of the previous challenge (brain age), allowing the submission of deep neural networks.
![]() |
This challenge was realized with the support of DATAIA Institute, in collaboration with University of Southern California (USC).
Alexandre Hutton, Sook-Lei Liew (Neural Plasticity & Neurorehabilitation Lab, Univ. of Southern California), Maria Teleńczuk, Swetha Shanker, Guillaume Lemaitre, François Caud, Alexandre Gramfort (Université Paris-Saclay, Institut DATAIA)
Introduction
Stroke is the leading cause of adult disability worldwide, and up to two-thirds of those affected suffer long-term disability. Large-scale neuroimaging studies have shown promise in identifying robust biomarkers (eg, measures of brain structure) of long-term stroke recovery after rehabilitation. However, analysis of large rehabilitation-related datasets is problematic because of barriers to accurate segmentation of brain lesions. Manually traced lesions are currently the gold standard for lesion segmentation on T1-weighted MRI, but they require anatomic expertise and are labor intensive. In addition, manual segmentation is subjective, with different graders producing different results.
Although algorithms have been developed to automate this process, the resulting lesion masks often lack the precision necessary to make them reliable information. Newer algorithms that use machine learning and deep learning techniques are promising avenues, but they require large and diverse datasets for training and testing and development of generalizable models. In this challenge, training can be performed on our public ATLAS 2.0 dataset, and testing is performed with a private dataset consisting of multi-site data from the same sites as ATLAS 2.0.
This challenge was realized with the support of DATAIA Institute, in collaboration with CEA NeuroSpin.
Antoine Grigis, Benoît Dufumier, Edouard Duchesnay (Université Paris-Saclay, CEA, NeuroSpin), François Caud, Alexandre Gramfort (Université Paris-Saclay, DATAIA)
Introduction
Modeling brain development and maturation in the healthy population using Machine Learning (ML) from brain MRI images is a fundamental challenge. The biological processes involved are complex and highly heterogeneous between individuals, including both environmental and genetic variability between subjects. Therefore, large MRI datasets including subjects of very diverse ages are needed. However, these datasets are often multi-site (i.e., images are acquired in different hospitals or acquisition centers around the world) and this induces a strong bias in current MRI data, due to differences between scanners (magnetic field, constructor, gradients, etc.).
Therefore, this challenge aims to build i) robust ML models that can accurately predict chronological age from brain MRI while ii) removing non-biological information from MRI images. We designed this challenge in the context of representation learning and it encourages the development of novel ML and Deep Learning algorithms.
Specifically, aging is associated with gray matter (GM) atrophy. Every year, an adult loses 0.1% of his or her GM. We will attempt to learn a predictor of chronological age (real age) using features derived from MG on a population of healthy control participants.
Such a predictor provides the expected brain age of a subject. A deviation from this expected brain age indicates an acceleration or slowing of the aging process that may be associated with a pathological neurobiological process or a protective factor of aging.
The dataset is composed of images from various sites, from different MRI scanners and acquired under various conditions. In order to correctly predict the age of the participants, the site/scanner effect must be considered.
This challenge was realized with the support of DATAIA Institute, in collaboration with the Institut National de Recherche pour l'Agriculture, l'Alimentation et l'Environnement (INRAE) and École Nationale Vétérinaire d'Alfort (ENVA).
Julien Chiquet (MIA Paris-Saclay, Inrae), Pierre Gloaguen (MIA Paris-Saclay, AgroParisTech), Nicolas Jouvin (MIA Paris-Saclay), Patrick Bouthemy (SERPICO, Inria), Alain Truibil (MaiAGE, Inrae), Alline Reis (PASP, ENVA), François Caud, Alexandre Gramfort (DATAIA, Univ. Paris-Saclay)
This challenge consists of predicting the developmental status of bovine embryos seen at 8 days post-fertilization (daf). There are 8 different classes (labeled "A" through "H" in this challenge) corresponding to biological states ranging from alive ("A") to dead ("H").
The known labels are the developmental state of the embryos at 8 daf, however, it is very interesting to be able to predict this future state as soon as possible. The goal of this challenge is to predict these states between 1 and 4 daf (at the latest) and to be as accurate as possible with respect to the indicated labels. For this, you have access to 277 videos from our own database (INRAE), each composed of 300 snapshots taken every 15 minutes.