Seminar l IntheArt - Tobias Liaudat (Irfu/Dedip)
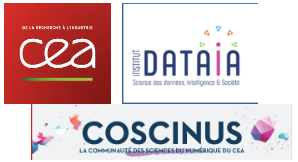
Evolutionary Bayesian uncertainty quantification with learned convex regularizers for radio interferometric imaging
The last decade has brought us substantial advances in computational imaging techniques for current and next-generation interferometric telescopes, such as the SKA. Imaging methods have exploited sparsity and the latest deep learning architectures with remarkable results. Despite good reconstruction quality, obtaining reliable uncertainty quantification (UQ) remains a common pitfall of most imaging methods. The problem of uncertainty quantification can be solved by reformulating the inverse problem in the Bayesian framework. The a posteriori probability density function provides a global understanding of uncertainties. However, calculating the posterior probability density function in high-dimensional environments is an extremely difficult task. Posterior probabilities are often calculated using sampling techniques, but these cannot yet be adapted to the high-dimensional parameters of radio imaging.
This work proposes a method to address uncertainty quantification in radio-interferometric imaging with data-driven (learned) priors for very high-dimensional settings. Our model uses an analytic physically motivated model for the likelihood and exploits a data-driven prior learned from data. The proposed prior can encode complex information learned implicitly from training data and improves results from handcrafted priors (e.g., wavelet-based sparsity-promoting priors). We exploit recent advances in neural-network-based convex regularisers for the prior that allow us to ensure the log-concavity of the posterior while still being expressive. We leverage probability concentration phenomena of log-concave posterior functions that let us obtain information about the posterior avoiding the use of sampling techniques. Our method only requires the maximum-a-posteriori (MAP) estimation and evaluations of the likelihood and prior potentials. We rely on convex optimisation methods to compute the MAP estimation, which are known to be much faster and better scale with dimension than sampling strategies. The proposed method allows us to compute local credible intervals, i.e., Bayesian error bars, and perform hypothesis testing of structure on the reconstructed image. We demonstrate our method by reconstructing simulated radio-interferometric images and carrying out fast and scalable uncertainty quantification.