Results of the call for internships M2 2020
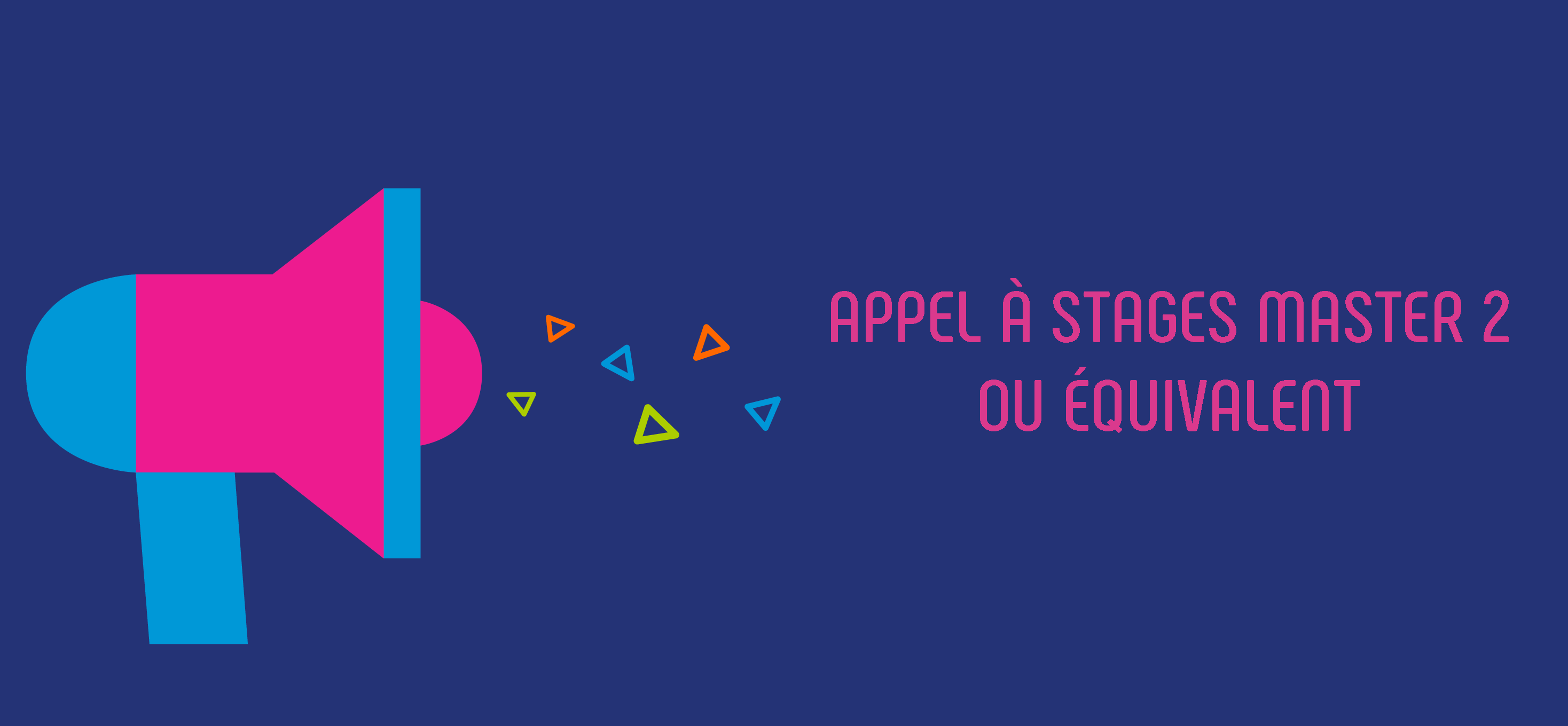
Results of the call for internships M2 2020
- Selected internships
- News on the same topic
The DATAIA Institute supports research in data science in the broadest sense - including computer science, mathematics and human and social sciences - through an annual call for Master 2 (or equivalent) internships.
This year's call, closed on November 12th 2020, has paid particular attention to interdisciplinary projects that involve the basic sciences.
Five internship topics have been selected, and will receive funding to host an intern for 4 to 6 months.
REASON
directed by Onofrio SEMERARO, Lionel MATHELIN (LIMSI, CNRS) and Michele Alessandro BUCCI (Inria)
The objective of the internship is to explore deep reinforcement learning (DRL) methods in the search for optimal control strategies in the context of fluid dynamics.
RobBSS4EEG
directed by Florent BOUCHARD, Matthieu JONCKHEERE, Frédéric PASCAL (L2S, CentraleSupélec) and Alexandre Gramfort (Inria)
The internship aims at formulating and solving a source separation problem under a hypothesis of ellipticity on the considered random variables, thus generalizing classical Gaussian models, and at applying the proposed method on electroencephalography data.
TDA4DRP
directed by Frédéric CHAZAL, Jisu KIM (Inria) and François COKELAER, Mathieu FERAILLE (IFPEN)
The proposed topic aims at implementing deep neural network approaches for the prediction of the permeability index of a rock from high-resolution 3D tomographic images, in particular by integrating features from Topological Data Analysis (TDA).
T-Rex
directed by Cédric MEHL, Thibault FANEY (IFPEN), and Michele Alessandro BUCCI, Marc SCHOENAUER (Inria)
The objective of the internship is to implement and evaluate a method based on the concept of "Physics Informed Neural Networks" (PINNS) (which consists in integrating physical a priori in the learning of a neural network) to solve a problem of reactive fluid mechanics.