The results of the DATAIA Institute's call for research projects 2018
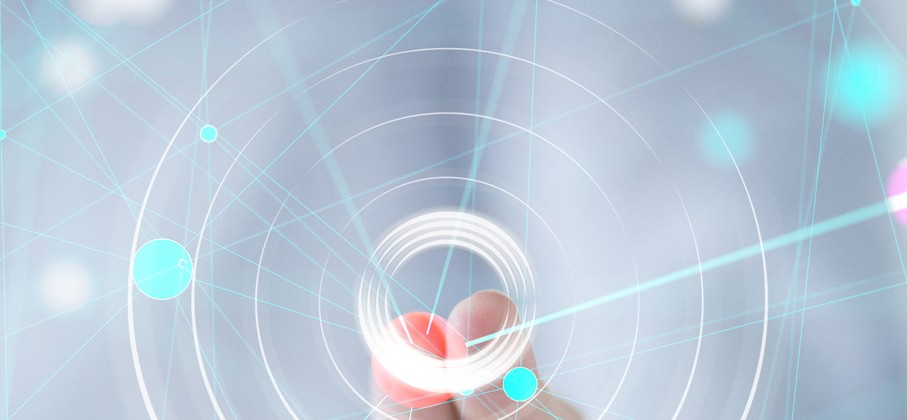
The results of the DATAIA Institute's call for research projects 2018
- News on the same topic
32 project proposals in at least one of DATAIA's four main challenges were submitted last February. Conditions of eligibility, these proposals had to rely on the collaboration of at least two people from two founding members of the DATAIA Institute not belonging to the same laboratory or to the same host institution. After studying the subjects received by the program committee, 12 of them were auditioned on April 9th.
The quality of the proposals and the richness of the topics allowed the selection committee to draw up a main list of 5 selected projects as well as a complementary list of 3 projects.
The prediction of renewable energy prosuming, the exploitation of data for job research assistance, the ethics in the interaction of conversational agents, the protection of personal data in a distributed model, the problem of missing data for the management, for example, of medical emergencies, here are the first subjects of study of the DATAIA Institute.
While waiting for more details on each of these subjects, discover the abstracts of the projects.
PEPER: prediction of prosummers with reinforcement deep learning
Today, the world of electric power is facing major structural changes: the use of electricity is constantly increasing and the climate challenges impose an increase in the share of renewable energy (solar and wind). Since these energies are by nature intermittent, uncertain and distributed over the entire territory, the centralized electricity grid is evolving towards a decentralized structure, made up of subsets that combine production, storage and consumption at the local scale (microgrids, building scale). They should cooperate to cover as much as possible, the needs on a larger scale (city, region, country). Prosumers (consumption behavior adaptation according to the energy produced and available) is then a key point to ensure the balance of the network. The efficient and cooperative energy management of such a system is based on the prediction of the behavior of the various actors of the network (producers and consumers), the exchange of data between them (cooperation), and this at different time and cost scales.
PEPER project will contribute to this interdisciplinary challenge.
The project will gather relevant data from different actors of the network, and exploit the deep learning techniques to develop algorithms for forecasting the production and consumption of each actor, then provide solutions for the cooperation between them . These algorithms integrate data of different natures in the past and present: geographical positions, meteorological measurements, production and energy consumption profiles, dynamic mobility of the populations at each position. They then produce consumption predictions, recommendations on consumption-related adjustments or recommendations on the complementarity between different geographical zones according to their production and consumption profiles.
Technically, this poses several scientific obstacles: choice of types of traces, or sources of most relevant data, choice of learning algorithms, cooperation technique between these algorithms to provide the expected results. The results of these learning techniques will then be compared to those of the mathematical tools currently used by the project's research teams. The partners will publish the algorithms developed in the PEPER project and their results will be deployed on a real physical testbed consisting of several new equipped buildings in Polytechnique zone and its neighborhood .
The project will start fall of 2018 and has a three years duration.
VADORE : Data Valorisation for Job Research
Our project focuses on unemployment in France. Unemployment has many causes, and they involve mainly factors limiting labor supply and demand. Unemployment can also hinge on the efficiency of the matching process that can pair demand with supply. In many cases there might be imperfections in the process, leading to the notion of frictional unemployment. Frictions in the labor market correspond to the case in which in a « micro labor market » there are both unfilled vacancies and jobseekers who would be willing to take them. These frictions in the labor market are related to imperfect information, and mostly due to the cost of collecting, processing and disseminating information; information asymmetry between employers and jobseekers; cognitive limitations of individuals that prevent them from scanning large numbers of job ads.
The central idea of the project is to mobilize all available information to improve the matching of jobseekers and vacancies. The project relies on the mobilization of the considerable body of information available at the Public employment Service in France on jobseekers and companies, some of which (textual data in particular) are still unexploited. This information will be used to develop two functionalities aimed at improving the matching process in the labor market for both jobseekers and firms: first a recommendation engine, and second an interactive personalized map for a jobseeker that will help her see the job market in her region/domain of competence and better appreciate her opportunities. One important aspect of the project is that the two functionalities will be evaluated using randomized control trial. The evaluation will be performed so as to identify the impact on inequalities in the labor market, e.g., whether using the tools leads to displacement effects.
Bad Nudge - Bad Robot ? : Nudge and Ethics in human-machine verbal interaction
Abstract: Our objectives in the DATAIA Project “NAD NUDGE BAD ROBOT” are to prove that it is easy to nudge children, adults and elderly people using social and affective robots and also to build metrics on bad nudges. We are all well-accustomed with the notion of ‘nudge’, as popularized by Richard Thaler, first behavioral economist to win the Nobel Prize (2017) and Cass Sunstein. Nudge theory attempts to study the use of positiv reinforcement techniques, indirect or tacit suggestions to influence decision making in groups or individuals in a way that is not forced. Social and affective robots begin to use affective computing technology with emotion detection and synthesis systems. These systems will use in a near future nudging strategies. When systems adapted to different cultures subtlety or overtly manipulate emotions and alter human behavior for commercial purposes, what kind of transparency and traceability will be present in these systems? Bad nudges, for example repetitive questions concerning private data, must be detected. Each of these are not — in themselves — enormous problems. But in aggregate, I would argue they constitute a form of nudging which is aggressive in nature. Another efficient bad nudging solution will be friend agents that give us advices for holidays, for health, for fashion to influence our choices. Two PHD theses, one in computer science, one in behavioral economy and law will be co-directed on this subject in France. Creating the reputation as a "Bad-Nudge-free" system for goods and services may be a winning long-run strategy in connexion with the P7008 IEEE working group on “Standard for ethically driven nudging for Robotic, Intelligent and Autonomous Systems”
GDPR and Personal Cloud: from Empowerment to Responsibility (GDP-ERE)
In a world disrupted by Artificial Intelligence and the exploitation of personal data, the role of individuals and the control of their data is a central issue in the new European regulation (GDPR), enforced on 25th May 2018, after the French Numeric Republic regulation adopted in 2016. Data portability is a new right provided under those regulations, introduced as a key element for powerful legal and technical tools. Building upon many projects such as Blue Button (health data) and Green Button (energy consumption data) in the US, MiData (energy, financial, telecommunications and retail data) in the UK or MesInfos in France, data portability allows citizens to retrieve their personal data from the companies and governmental agencies that collected them, in an interoperable digital format. Data portability thus gives the individual the ability to get out of a captive ecosystem, to regain control of his or her personal data towards empowerment and informational self-determination. It also opens to important societal benefits when individuals collectively decide to make their data available to public service missions, citizen actions (e.g., « in vivo » tests of an algorithm presenting risks of bias) or a scientific studies (e.g., epidemiological surveys). Finally, data portability represents a new vector of development for innovative and virtuous personal data economy beyond the existing de facto monopolistic positions.
The consequence of this new right is the design and deployment of technical platforms, commonly known as Personal Cloud. Individuals are thus able to retrieve all their personal data from different data silos and group them in a single system, with the ability to control all access and usage in favor of innovative services. Yet, managing all the « digital assets » of an individual in one platform obviously raises security and privacy issues. But personal cloud architectures are very diverse, ranging from cloud based solutions where millions of personal cloud are managed centrally, to self-hosting solutions where the individual installs a personal server on his or her own own equipment. These architectural choices are not neutral both in terms of security (risk of massive attacks for centralised solutions versus lack of IT expertise of individuals for decentralised solutions) and from the point of view of the chain of liabilities. This last point particularly deserves to be studied. For example, considering a context of self-hosting under the angle of liabilities imposes to reexamine the role of every actor involved (controller, processor, third party) and redefine their respective prerogatives and obligations. The GDP-ERE project tends to solve those issues in an interdisciplinary approach by the involvement of jurists and computers scientists. Two main objectives are sought under this project: (i) analysis of the effects of the personal cloud architectures on legal liabilities, enlightened by the analysis of the rules provided under the GDPR; (ii) proposals of legal and technological evolutions to highlight the share of liability between each relevant party, and create adapted tools to endorse those liabilities.
GDP-ERE project builds on an existing collaboration between jurists researchers and computer scientists. Researcher activities are supported by some national authorities (including the French data protection authority, CNIL) and personal cloud platform providers.
MissingBigData: missing data in the big data era
“big data”, often observational and compound, rather than experimental and homogeneous, poses missing-data challenges: missing values are structured, non independent of the outcome variables of interest. We propose to use more powerful models that can benefit from the large sample sizes, specifically autoencoders, to impute missing values. To avoid biasing conclusions, we will study multiple imputation and conditions on the dependencies in the data.
Our project will enable proper causal interpretations of the risk factors despite data missing not at random. We seek an operational solution, from the methodology to the implementation, that integrate the diversity of data and of questions while dealing with larger data. Indeed, combining predictive models with causal inferences is classic and existing methodologies focus on one or other of the problems, though the missing-data methodology impacts the whole process. We will also depart from classic studies by considering multiple types of missing data and MNAR on multiple variable. This will be a first, but seems feasible in view of the theoretical results of Mohan and Pearl (2018 ).
Smart Lawyer: Rating Legal Services in the Courtroom
On 11 May 2017, the French Cour de cassation handed down its decision n° 561 (16-13.669) on which it acknowledged the importance of online services and platforms offering comparative assessments of lawyers and law firms, including through rankings and ratings, for the protection of consumers of legal services. However, the Court also affirmed that such services must ensure a certain level of quality: “[il] leur appartient [...], dans leurs activités propres, de délivrer au consommateur une information loyale, claire et transparente”. Indeed, the Court held that providers of ratings, which are designed to inform the behaviour and decisions of consumers, should ensure that such information is loyal, clear and transparent. Unfortunately, to date, providers of these services rely mostly on anecdotal evidence as well as on perception and self-reported data. The lack of reliable information about the quality of legal services delivered by lawyers in the courtroom is a worrying and widespread phenomenon in all jurisdictions across the European Union, but also in other jurisdictions such as the United States and Canada. This project aims to fill this gap by combining legal expertise with data science research. It seeks to develop a meaningful and reliable measurement tools of legal performance in the courtroom that can help improving access to justice and the quality of legal services, while also helping law firms assess the performance of lawyers and the quality of jurisdictions.
HistorIA : Large historical databases. Data mining, exploration and explicability
The development of computational approaches to social sciences has stimulated new ambitious projects in historical research. However, the drastically new ways of dealing with historical sources come with high criticism and distrust from many historians who feel they might lose an essential contact with their work material.
Our project, HistorIA, gathers researchers from history, computational social sciences and information visualization. Its aim is to develop large data bases from historical sources with data mining analyses, along with iterative exploration tools, putting the main focus on the explicability of algorithms with visualization interfaces based on progressive analysis. The workflow will involve iterative steps of algorithm design by computer scientists and visual exploration by historians.
StreamOps: Next Generation Streaming Platform: System Requirements and Research Directions
In the last few years, streaming platforms have become increasingly popular.
This trend has been driven by requirements to quickly process never-ending flows of human-generated data or physical measurements, and supported by huge efforts in the open-source community (sometimes coupled with initiatives from web-oriented companies). Beyond the need to offer a cross-fertilized robust and scalable streaming platform to researchers, the current context poses new challenges for such a tool: in particular we will present the idea of a privacy-aware and accountable streaming platform, and develop related implications in terms of algorithms design.